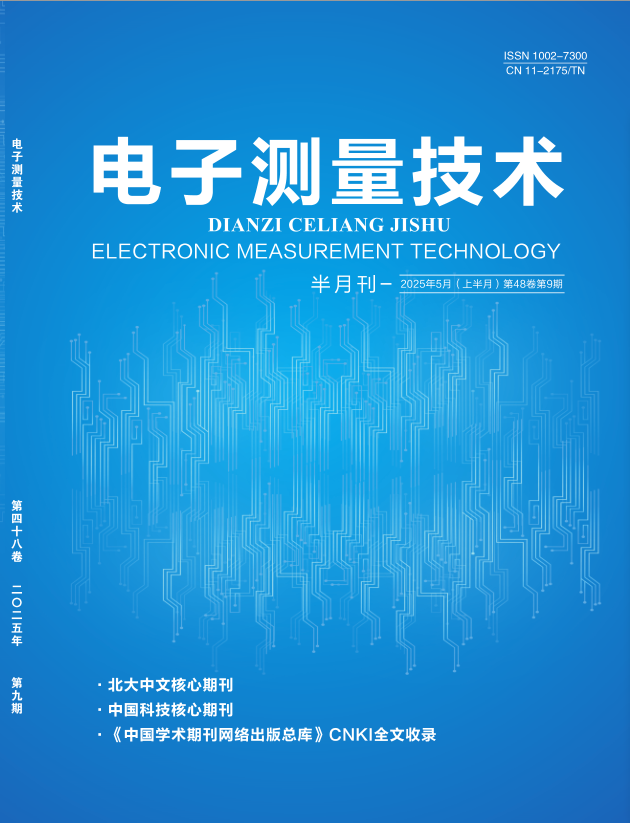
Editor in chief:Prof. Sun Shenghe
Inauguration:1980
ISSN:1002-7300
CN:11-2175/TN
Domestic postal code:2-369
- Most Read
- Most Cited
- Most Downloaded
Wang Yi , Qin Chao , Xu Zhenbang
2025, 48(9):1-8.
Abstract:To meet the demand for non-contact synchronous measurement of the large-range six-degree-of-freedom pose of a parallel robot′s moving platform, a pose measurement system composed of a laser and visual components is proposed. The system utilizes image processing technology to output the two-dimensional coordinates of light spots on the detection surface, achieving precise pose measurement of parallel robot platforms through the cooperation of lasers and vision components. Subsequently, the solution method of the measurement system is studied, and the pose of the target is derived based on analytical geometry methods. Finally, experimental tests are conducted using a high-precision parallel robot. The results indicate that the system achieves a root mean square error of less than 0.1 mm in position measurement and less than 0.07° in attitude measurement. The average relative errors of position and attitude measurements are 0.76% and 2.56%, respectively. This research provides a novel and effective solution for large-stroke pose measurement technology, with broad application prospects in robotic motion control, precision manufacturing, and scientific experiments.
Lu Zhaoqing , Wang Hongwei , He Li , Si Panzhao , Chen Yaohua
2025, 48(9):9-18.
Abstract:In unknown or hazardous environments (such as emergency rescue and disaster relief), traditional navigation methods struggle to achieve specific target navigation due to the inability to obtain prior maps and location information. This paper proposes a target-driven autonomous navigation method for mobile robots based on dueling double deep Q network (D3QN). The cross-modal fusion module of this method dynamically weights and integrates features from different modalities, effectively consolidating observational data while fully capturing environmental information. This significantly enhances the capability to perceive the environment.Building on this, a general target-driven navigation approach is designed, where YOLOv5 is used to recognize specific targets (such as flames or smoke) and obtain their locations. The identified target locations are used to replace predefined waypoints in deep reinforcement learning-based navigation, enabling autonomous navigation to specific targets. Simulation results show that the proposed method has significant advantages in the navigation success rate and other indicators. In simple, complex, and dynamic scenarios, the success rates increased by 9%, 27%, and 38%, respectively. Moreover, the model trained in a simple simulation environment can be directly deployed in more complex simulation environments and real-world scenarios, exhibiting strong generalization capability.
Li Qiang , Ma Chao , Huang Min
2025, 48(9):19-26.
Abstract:Aiming at the characteristics of complex features in bearing fault signals, a bearing fault determination method combining the attention mechanism and multi-scale residual convolutional network is proposed. The model combines the powerful feature extraction capability of convolutional neural network (CNN) and the adaptive weighting capability of the attention mechanism, which can effectively deal with the complex features in the bearing fault signal. The model employs a multi-scale convolutional layer, which captures the multi-scale features of the signal through different sizes of convolutional kernels, which helps to recognize different types and severities of faults. Meanwhile, the residual structure is introduced to effectively integrate the features extracted by multilayer convolution through the cooperative decision-making mechanism of high-dimensional and low-dimensional features, which enhances the model′s ability to perceive the key information and reduces the problems of gradient vanishing and feature redundancy in the training of the deep network, so as to ensure the stability and accuracy of the model. The fusion of attention mechanisms (e.g., SEBlock and ECABlock) enables the model to adaptively focus on more important feature channels, which further improves the diagnostic performance. The experimental results show that the model can achieve high-precision diagnosis under various fault modes, demonstrating its potential application in intelligent maintenance and fault warning systems.
Wang Yu , Peng Xincun , Zou Jijun , Deng Wenjuan , Jiang Linlin
2025, 48(9):27-35.
Abstract:The positive electron affinity potassium cesium antimony photocathode has driving laser wavelength is longer (532 nm), high quantum efficiency, long life, fast response time, in the field of electronic source has broad application potential, but limited by the photoelectric characteristics of the material itself, effective transport distance is very short, not enough to absorb all the incident light driven laser energy, and quantum efficiency is also affected. Therefore, this article introduced the Fabry-Perot cavity structure into the photocathode, to improve the effective absorption of incident driven laser, through the time domain finite difference method simulation study, make the active layer potassium cesium antimony light absorption reached 90%, and the reflective layer silver and dielectric layer silicon nitride were prepared and verified, predicted the quantum efficiency is 11.17%, to meet the requirements of high brightness, high frequency electron source in the future.
Yuan Haoren , Zhou Fuqiang , Sun Jianghong
2025, 48(9):36-43.
Abstract:In practical applications, the expansion state observation in self disturbance rejection controllers faces the problem of inability to guarantee estimation and compensation accuracy when faced with significantly changing load disturbances. A scheme is proposed to improve the estimation and compensation accuracy by compensating the load disturbance observed by the load torque observer to the extended state observer. The load torque observer is designed using angle data that can be directly measured in the motor as a known quantity, avoiding the problem of introducing differential errors by using the speed as a known quantity. The simulation and experimental results show that the improved linear active disturbance rejection controller reduces the maximum speed adjustment by 7.24% compared to the traditional linear active disturbance rejection controller, and has better anti load disturbance performance while ensuring the adjustment speed.
Wang Jiawei , Fu Shengwei , Huang Haisong
2025, 48(9):44-55.
Abstract:The rime optimization algorithm (RIME) is an intelligent optimization algorithm inspired by the natural growth process of rime. It demonstrates strong optimization capability by employing a soft rime strategy for global search and a hard rime strategy for local exploitation. However, RIME suffers from slow convergence and a tendency to fall into local optima during applications. To address these issues, this paper proposes an improved rime optimization algorithm (IRIME). First, a dynamic centroid guidance strategy is introduced in the early stages of the algorithm, significantly enhancing convergence speed. Second, an improved differential mutation operator is incorporated into the later iterations to effectively reduce the risk of the algorithm becoming trapped in local optima. Additionally, a novel centroid boundary adjustment strategy is designed to enable collaborative optimization of accuracy and efficiency by deeply exploiting population information. Experiments conducted using the CEC2017 benchmark set demonstrate that IRIME outperforms PPSO, AGWO, HPHHO, RIME, and SRIME in optimization performance. Furthermore, IRIME is applied to the three-dimensional path planning problem for UAVs. The results indicate that IRIME provides substantial improvements in solution quality, convergence stability, and computational efficiency, offering an effective solution for complex engineering optimization problems.
Qin Lunming , Zhang Yunqi , Cui Haoyang , Bian Houqin , Wang Xi
2025, 48(9):56-64.
Abstract:To address the issues of decreased detection accuracy and difficulty in small object recognition caused by blurred traffic signs in extreme weather conditions such as rain, fog, and snow, this paper proposes a traffic sign detection algorithm based on an improved RT-DETR. First, data augmentation is applied to the TT100K dataset under simulated extreme weather conditions to enhance the model′s ability to recognize traffic signs in these environments. Second, the Ortho attention mechanism is introduced into the backbone network, which uses orthogonal filters to reduce feature redundancy and prioritize essential channel information, thereby improving the model′s detection accuracy for small objects. Additionally, a high-level screening-feature pyramid network (HS-FPN) replaces the cross-scale contextual feature mixer (CCFM) in the original model. HS-FPN filters and merges low-level feature information using high-level features, enhancing the model′s detection accuracy for low-contrast and blurred targets in extreme weather conditions. Experimental results show that the proposed improved algorithm achieves an average detection accuracy of 87.84%, an improvement of 2.37% over the original RT-DETR model, while reducing the parameter count to 18.22 M, an 8.4% reduction compared to the original model. The model demonstrates higher accuracy in recognizing small objects and targets under extreme weather, contributing significantly to passenger safety.
Han Dongsheng , Lang Yuhang , Huang Liyan
2025, 48(9):65-74.
Abstract:In distributed IoT application scenarios such as environmental monitoring, nodes often have different priorities due to the different regional importance of node monitoring and the amount of data collected. The dynamic change of node priority will make the UAV frequently replace the target node of data acquisition, resulting in prolonged task completion time and unwarranted waste of energy. Therefore, we propose a joint optimization algorithm of UAV task completion time and energy consumption based on DDQN for distributed IoT application scenarios with dynamic priority of nodes. During the training process, the UAV learns the optimal strategy under the constraints of task completion time, energy consumption and avoiding node data overflow. The simulation results show that compared with the maximum priority strategy and greedy strategy, the task completion time of the proposed algorithm is reduced by 9.2% and 15.1% respectively, and the energy consumption is reduced by 10% and 16.3% respectively. Compared with the DQN method, the proposed algorithm converges faster and the training process is more stable.
Liu Bo , Wu Songrong , Fu Cong , Wang Shaowei , Zhang Chi
2025, 48(9):75-83.
Abstract:The state of charge (SOC) of the battery is one of the core parameters for managing lithium batteries in electric vehicles. This paper proposes a lithium battery SOC estimation model based on an improved white whale algorithm optimized BiTCN-BiGRU. Firstly, a SOC estimation model combining bidirectional time domain convolutional network (BiTCN) and bidirectional gated recurrent unit (BiGRU) is constructed. Then, the beluga whale optimization (BWO) is used to optimize the hyperparameters of the BiTCN-BiGRU model to fully leverage the advantages of the combined network model. Improvement strategies are introduced in the exploration and whale fall stages of traditional BWO to solve the problem of traditional BWO easily falling into local optima and slow convergence speed. Finally, the performance of the improved SOC estimation model was verified based on the open-source lithium battery charging and discharging dataset. The results showed that under standardized urban cycling conditions at three temperatures, the improved white whale algorithm optimized the BiTCN-BiGRU model SOC estimation with an average absolute error of 0.428% and a root mean square error of 0.38%, which can be well applied to lithium battery SOC estimation.
Lian Xiaoqin , Liu Chunquan , Gao Chao , Deng Ziqian , Wu Yelan
2025, 48(9):84-93.
Abstract:In recent years, motor imagery (MI) has attracted significant attention in the fields of assistive healthcare and human-computer interaction. However, the classical common spatial pattern (CSP) feature extraction method is mainly based on calculating covariance matrices (CM) from time-domain signals, making it susceptible to noise and artifacts while failing to fully exploit the spectral information of electroencephalogram (EEG) signals. This limitation reduces classification accuracy and stability. To address this problem, this study proposes a MI-EEG classification algorithm based on regularized spectral covariance matrix (RSCM) and Riemannian space. Firstly, the preprocessed EEG signals undergo fast Fourier transform (FFT) to compute spectral covariance matrices, followed by ridge regularization. Then, the regularized matrices are mapped into the tangent space for geodesic filtering and projected back to the Riemannian space for CSP feature extraction. Finally, classification is performed using SVM. Experimental results demonstrate that, on BCI Competition IV datasets 1 and 2a, the proposed method achieves an average binary classification accuracy of 86.95% and 81.48%, respectively, outperforming traditional CSP by 7.44% and 9.57%. In the four-class classification task on BCI Competition IV dataset 2a, it reaches 74.23%, representing a 14.10% improvement over traditional CSP. These findings indicate the effectiveness of the proposed method in MI-EEG classification.
Chen Yinchao , Wang Tao , Wang Kai , Liang Zhaoxin , Wang Rui
2025, 48(9):94-99.
Abstract:Brushless dc motors (BLDCs) are widely used in various industrial fields. Failures in critical applications can lead to significant economic losses or even casualties, highlighting the importance of fault diagnosis research. BLDCs often operate under varying conditions, leading to the differences between the source and target domains used in data-driven models. While domain adaptation methods are commonly used to address this problem, they require access to the target domain during training, complicating model deployment. To overcome this, we propose the angular domain data mixed domain generalization network (ADMDG). This method leverages multiple source domains from different BLDC operating conditions to learn domain-generalized knowledge, enabling effective generalization to unseen target domains and allowing for a single training process to support multiple deployment scenarios. ADMDG employs an angular domain current resampling technique to convert timedomain currents into angular-domain currents, mitigating the impact of varying conditions. A convolutional neural network-based fault diagnosis model is constructed, and advanced data augmentation techniques, Mixup, are used to enhance model generalization. Extensive BLDC fault experiments demonstrate the superior domain generalization performance of the proposed method compared to other state-of-the-art approaches.
Cai Bingbin , Huang Danping , Zhang Haotian , Liao Shipeng
2025, 48(9):100-110.
Abstract:To address the current challenges of difficult image acquisition and slow detection for bearing defects, a single-station online detection system for full surface defects of bearings based on machine vision has been developed. The system utilizes a line-scan camera in conjunction with an optical system to efficiently capture images of the entire surface of the bearings. The defect detection network model constructed is based on ConvNeXt as the feature extraction backbone, employing feature fusion to supplement feature information, it then undergoes lightweight modifications. Additionally, a multi-task learning training approach is adopted, enabling the model to effectively process feature information at various levels, thereby significantly enhancing its performance in bearing surface defect detection tasks. Experimental results show that compared to ConvNeXt-Tiny, the proposed deep learning model achieves a 4.14% improvement in detection accuracy, reaching 99.02%. When relying on CPU for computation, the average detection time per bearing by the single-station full surface defect online detection system is 0.735 seconds. This system is characterized by its compact size, low cost, and ability to meet the requirements for online detection of bearing surface defects, showcasing promising application prospects.
Li Jingchen , Chen Jian , He Libo
2025, 48(9):111-118.
Abstract:To meet the requirement of testing whether the 1553B interface function of the single machine of the on-board payload is normal, a software and hardware design scheme of a 3U PXIe peripheral module based on FPGA is proposed. The PXIe control module can configure the 1553B module through the PXIe bus and control and transmit the simulation and test data of the 1553B signal of the payload. This design fully simulates the real circuit and electrical characteristics of the 1553B interface of a certain satellite′s on-board computer, and realizes the BC and BM functions of the bus as required. Through the B-6131 bus protocol chip, two 1553B bus interface lines with the above functions are configured, which are highly consistent with the actual application scenarios. Through the operation of the on-board platform test software, one 1553B channel working in BC mode can send custom messages, and can also start a period of broadcasting, long loopback test and other semi-automated tests to achieve adaptive bus testing. All messages appearing on the 1553B bus will be captured by another bus channel working in BM mode, thereby realizing the monitoring and storage function of all bus data.
Qin Dong , Ren Xiaoming , Ye Zhou , Chen Jian
2025, 48(9):119-128.
Abstract:To improve traffic monitoring efficiency, particularly in situations where the field of view of fixed road cameras is limited, a drone-based traffic monitoring system using the PLCnext platform is proposed. This system integrates YOLOv9 deep learning technology, enabling two UAVs equipped with cameras to cooperate with the PLCnext platform. This collaboration provides broader coverage and more flexible monitoring capabilities for real-time vehicle surveillance. To enhance detection accuracy in low-resolution aerial images, a new model, YOLOv9s-SPDADown-LSK, is introduced. The model utilizes a SPD layer to improve image detail retention, optimizes the downsampling process with the ADown module, and incorporates the LSK attention mechanism in the backbone network to enhance feature extraction. Experimental results indicate that the system achieves an image processing delay of approximately 80 ms, with the modified model reaching mAP@0.5 and mAP@0.5:0.95 values of 96.3% and 82.7%, respectively. The detection accuracy stands at 97.2%, significantly reducing false positives and false negatives, thereby validating the system′s feasibility and the effectiveness of the proposed algorithm.
2025, 48(9):129-139.
Abstract:As meteorological observations evolve towards socialization and intelligence, the existing ground-based automatic weather stations can no longer meet the new demands due to their split design, large volume, high power consumption, and cost. This paper designs a multi-element modular automatic weather station. This weather station uses modular composition and digital sensor technology to flexibly observe meteorological elements such as temperature and humidity, air pressure, particulate matter 2.5, wind speed, and wind direction. This paper employs a band-pass filter designed with multi-channel negative feedback circuits to address the severe environmental interference affecting ultrasonic anemometers. This approach reduces the scale of the ultrasonic signal conditioning circuit and improves the quality of the ultrasonic pulse signals. Moreover, an adaptive filter algorithm with a sliding window size adjustment factor is introduced to reduce signal environmental interference. The experimental results show that the digital sensors used in the designed automatic weather station meet the observation requirements. Specifically, the wind measurement module has a maximum wind speed error of 14.8% and a maximum wind direction error of 11.78%. These figures indicate that the new weather station achieves high accuracy in wind measurements and demonstrates stable performance.
Wu Yuqian , Zhang Xiuzai , Li Jingxuan
2025, 48(9):140-148.
Abstract:The essence of cloud image prediction is spatiotemporal sequence prediction. Deep learning-based spatiotemporal sequence prediction algorithms can be categorized into three frameworks: SISO, MIMO and MISO. Based on the characteristics of cloud image movement, designed a cloud image prediction algorithm under the MISO framework, combining the features of both MIMO and SISO frameworks, called the implicit autoregressive spatiotemporal channel aggregation prediction (IASCACP) algorithm. To address the issue of image correlation loss in MIMO models and excessive error accumulation in SISO models, introduce an implicit autoregressive encoder-decoder. This encoder-decoder endows the model with certain recursive properties through an implicit autoregressive structure to capture spatiotemporal correlation information in sequences. Additionally, a masking and true mapping module is used to reduce error accumulation from recursion and enhance model robustness. To tackle issues such as instability and nonlinearity in cloud movement, we designed a spatiotemporal channel aggregation predictor. This module effectively aggregates multi-order spatiotemporal interaction information and performs adaptive channel reallocation to reduce feature redundancy. The algorithm was tested on the MovingMNIST dataset and the FY-4A satellite cloud image dataset. Experimental results show that this algorithm effectively improves the shortcomings of MIMO and SISO models and achieves higher prediction accuracy compared to other models, demonstrating its reliability and effectiveness in the field of cloud image prediction.
Zhou Ruoxuan , Zhang Ruiqian , Chen Yong , Yuan Xuhao , Qin Huijun
2025, 48(9):149-155.
Abstract:The traditional ORB-SLAM3 system demonstrates excellent performance in static environments, however, the presence of dynamic features introduces unnecessary noise, leading to errors in feature matching and inaccuracies in camera pose estimation. Existing dynamic SLAM algorithms face challenges in comprehensively identifying potential dynamic features, resulting in missed detections or false positives and consequently degrading localization accuracy. To tackle these issues, the semantic segmentation network Deeplabv3+ and the Lucas-Kanade optical flow method are incorporated into the tracking thread of ORB-SLAM3. Specifically, the backbone network of Deeplabv3+ is replaced with Mobilenetv3 to enhance the precision of semantic segmentation. Semantic segmentation is then used to obtain a mask of potential dynamic objects, which is employed to preliminarily filter out dynamic feature points. The remaining feature points undergo LK optical flow calculation, with the average optical flow error serving as a threshold to prevent the insufficient number of static feature points from causing pose estimation failure. In comparison to the original ORB-SLAM3, the improved algorithm in this study achieves an average localization accuracy improvement of 47.92% on the high-dynamic sequences of the TUM dataset. Furthermore, among existing advanced dynamic SLAM algorithms, the proposed method achieved the highest localization accuracy on the Walking_static sequence of the TUM dataset.
2025, 48(9):156-167.
Abstract:In response to the issues of missed and false detections in transmission line defect detection tasks caused by varying target scales, complex backgrounds, and occlusion, where existing object detection algorithms struggle to maintain detection accuracy while ensuring real-time performance, an improved YOLOv10-based UAV transmission line defect detection algorithm, TLDDet, is proposed. First, a faster C2F with attention module(FC2FA) incorporating context anchor attention is designed to enhance feature integration capabilities while reducing the model′s parameters. Then, an attention-based intrascale feature interaction module (AIFI) based on multi-head self-attention is used to improve the model′s detection performance for small objects by enhancing the representation of high-level semantic information in the feature map, thereby increasing the detection accuracy. Finally, an occlusion-aware attention detection head (SEAM-Head) is designed to reduce feature loss caused by occlusion. Experimental results show that the proposed TLDDet reduces the parameters by 33% and the computational cost by 30% compared to the original YOLOv10s model, while improving precision, recall, and mean average precisionfor various transmission line defects by 4.3%, 2.4%, and 3.7%, respectively. The detection speed reaches 143 FPS, and comparative experiments with other real-time detection algorithms demonstrate superior model performance, making TLDDet more suitable for transmission line defect detection tasks.
Zhang Guojing , Li Chengjia , Han Jingwei , Huang Man
2025, 48(9):168-176.
Abstract:Pupil localization plays a crucial role in human-computer interaction and biomedical computing applications. Currently, many sophisticated pupil localization algorithms are designed to detect and locate the pupil position using one single image. However, pupil movement is a continuous process. Therefore, when the pupil position cannot be accurately detected and located in one current frame, the pupil position can be inferred by combining information of previous frames. This approach can more effectively handle difficult and challenging situations such as reflections, pupil occluded by eyelashes and blinks, as well as off-center pupil positions and motion blur. Consequently, it can significantly improve the accuracy and robustness of pupil detection, decreasing localization errors. To address these challenges, propose a pupil detection algorithm based on deep learning using multiple consecutive images. This algorithm enhances the standard Unet encoder-decoder structure by incorporating multi-frame information from continuous eye tracking scenes for improved pupil detection. By combining convolutional neural networks with convolutional long short-term memory networks and a convolutional block attention module, we introduce a hybrid semantic segmentation network. Experiments on a large-scale dataset demonstrate that the proposed method outperforms existing pupil detection algorithms, achieving a mean intersection over union score of 96.78% and a root mean square error value of 3.83, especially in challenging situations.
2025, 48(9):177-188.
Abstract:Aiming at the problems of low accuracy of small target detection, large number of parameters, as well as misdetection and leakage in tile surface defect detection, an improved tile surface defect detection algorithm, YOLOv9s-SEFN, is proposed. Firstly, the SPNet multi-scale feature fusion module is designed in this study to effectively improve the model′s detection of small defects on the tile surface by enhancing the network′s capability of capturing and fusion of multi-scale feature expression; second, the ECG lightweight fusion module is designed to reduce the computational and parametric quantities to achieve lightweighting; then, the frequency adaptive dilation convolution (FADC) is introduced to improve the accuracy of small defects detection on tiles by adaptively adjusting the dilation rate and frequency selection; and lastly, a new loss function, NWD-EIOU, is designed to improve the accuracy of small target localization by combining EIOU and NWD. The experimental results show that compared with the original YOLOv9s detection algorithm, the improved YOLOv9s-SEFN algorithm performs better on the self-built experimental dataset, with the mAP@0.5 raised to 93.2%, an improvement of 3.5%; the recall rate is raised by 4.96%; the amount of parameters is reduced by 2.3%; and the amount of floating-point arithmetic is reduced by 4.0%, which is able to satisfy the needs of tile surface defect detection.
Zhang Zheng , Zheng Yingqiao , Tian Qing
2025, 48(9):189-197.
Abstract:Laser range gating technology can break through the limitations of traditional imaging in complex environments such as rain, snow and fog, low light and inverse glare, but the generated gated image is a lowquality grayscale map, which lacks color information and is difficult to distinguish between the subject and the background, so super-resolution reconstruction technology is needed to focus on the reconstruction of edge information and spatial details to improve the visual effect. Due to the lack of color and rich texture information in the gated image, the traditional feature extraction method is prone to redundant features, which affects the reconstruction efficiency. In order to solve the above problems, this paper proposes a bi-aggregation deep feature extraction network. Firstly, shallow feature extraction was carried out by spatial and channel reconstruction convolution (SCConv) to improve the information content and solve the redundancy problem. Secondly, a new deep feature extraction module was designed to enhance the capture of the edges and details of the gated image. Finally, continuous nearest neighbor interpolation and convolution operations are used for image reconstruction, which effectively avoids the problem of artifacts. Experiments on the gated image dataset show that compared with the baseline DAT algorithm, the PNSR index of the proposed method is increased by 0.19 dB, 0.12 dB and 0.04 dB under the condition of 2 fold, 3 fold and 4 fold resolution degradation, respectively, and the SSIM is increased by 0.000 5, 0.000 8 and 0.001 0, respectively, and the results show that the proposed method can achieve better visual effects.
Editor in chief:Prof. Sun Shenghe
Inauguration:1980
ISSN:1002-7300
CN:11-2175/TN
Domestic postal code:2-369