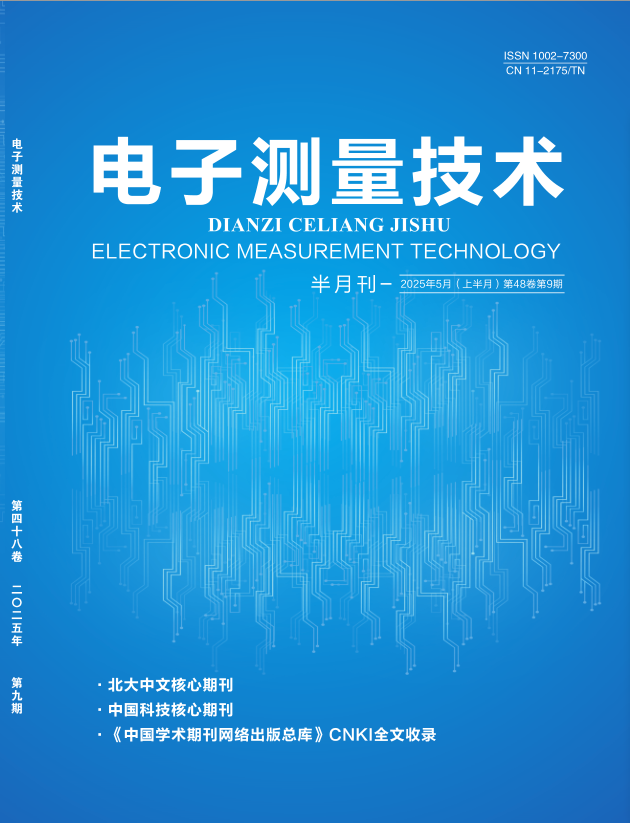
Editor in chief:Prof. Sun Shenghe
Inauguration:1980
ISSN:1002-7300
CN:11-2175/TN
Domestic postal code:2-369
- Most Read
- Most Cited
- Most Downloaded
Zeng Qi , Zhang Hao , He Chuan , Huang Xiaobing
2025, 48(8):1-9.
Abstract:With the development of renewable-energy-dominated power system, current differential protection, a key technology to ensure the safe operation of power systems, has attracted widespread attention regarding its reliability. However, the influence of noise interference in the process of information transmission is often ignored in the existing studies when discussing current differential protection, which may lead to the failure of protection action. Deeply analyzes the influence of complex noise disturbance composed of Gaussian white noise and impulse noise on the operation characteristics of current differential protection, based on the ρ-plane theory, and proposes a new sector criterion. The sector criterion can flexibly set the minimum phase difference ϕop and the minimum amplitude ratio R of the current at both ends when the line is fault-free to achieve a dynamic braking area, which effectively improves the anti-interference ability and operation reliability of the system under noise disturbance. Compared with the traditional circular criterion, the proposed sector criterion exhibits superior performance in resisting mal-operation and refusal operation under different signal-to-noise ratio, which can be validated by theoretical analysis and simulation experiments. The sector criterion reduces the mal-operation rate by about 50% compared to traditional criterion, and can ensure that both the mal-operation rate and the refusal operation rate are far less than 10-5, even under complex noise disturbances with a low signal-to-noise ratio (30 dB). It provides a high-reliability solution for current differential protection in the distribution network, which has important theoretical and practical significance for ensuring the safe and stable operation of the new power system.
Fan Ziyan , Zhu Yaodong , Zhao Weilan , Li Xuanyi
2025, 48(8):10-17.
Abstract:Dysarthric speech arises from neurological disorders that cause motor impairments in the articulatory organs, resulting in abnormal pronunciation and prosody, which pose significant challenges to traditional ASR systems. To address these issues, this paper proposes an innovative algorithm that combines a multi-level representation fusion decoding strategy with hotword boosting technology. Built upon the Transformer-based encoder-decoder architecture, the approach improves the conventional single-view decoding method by introducing multilevel representation fusion. This is achieved through three distinct fusion strategies, which effectively enhance the model′s ability to comprehend complex sentences and contextual information. Additionally, to further improve the recognition accuracy of dysarthric speech, hotword boosting is integrated into the beam search decoding process to assign higher weights to key terms. The results demonstrate that the proposed method significantly reduces the WER compared to other baseline models. Specifically, compared to the Whisper baseline model, the WER on the UASpeech dataset decreased from 38.31% to 27.18%, and on the TORGO dataset, it decreased from 16.38% to 12.67%. This highlights the effectiveness of the proposed method in improving the accuracy of dysarthric speech recognition.
Huang Huibao , Chen Rong , Chen Jiankang , Luo Chong
2025, 48(8):18-23.
Abstract:Reservoir dams, as critical infrastructures for flood control, hydropower generation, and agricultural irrigation, pose significant risks if breached, making regular safety monitoring essential. However, monitoring data often contains anomalies due to environmental factors, system malfunctions, and abnormal behaviors of the monitored objects. Detecting these anomalies is vital for effective data analysis and early risk identification to ensure the dam safety. Existing anomaly detection methods typically focus on gross outliers and overlook subtle, gradual anomalies. This paper proposes a gradual anomaly detection method based on Deep Support Vector Data Description(DSVDD). The method constructs multi-dimensional monitoring parameters as samples and trains an autoencoder using DSVDD to map the input data to a compact hypersphere. The anomaly score is derived from the distance of the input sample from the center of the hypersphere. The proposed method is validated using laser alignment data from a high core-wall rockfill dam, and the results demonstrate its superior performance compared to other methods.
Su Jiuzheng , Hu Wenshan , Lei Zhongcheng , Li Kunjie , Liu Binbin
2025, 48(8):24-33.
Abstract:In order to solve the problems of low prediction accuracy of the traditional rolling force model and the dung beetle optimizer(DBO) algorithm′s tendency to fall into local optimal solutions, a rolling force prediction model based on the improved dung beetle optimisation algorithm combined with the self-attention(SA) mechanism for long and short-term memory(LSTM) networks is proposed. An improved dung beetle optimizer(IDBO) algorithm is obtained by adding the golden sine algorithm and dynamic weight coefficients and introducing the Circle chaotic mapping, and by combining the LSTM network with the SA mechanism, the IDBO-SA-LSTM cold rolling force prediction model is established, and compared with other models. Six different benchmark functions are used for testing, and simulation experiments show that IDBO algorithm has faster convergence speed and optimization accuracy than the sparrow search algorithm, the dung beetle optimization algorithm, the grey wolf search algorithm and so on. The rolling force prediction experiments are carried out using 6554 field operation data of a two-stand cold rolling unit, and the results show that the prediction error indexes of the IDBO-SA-LSTM algorithm are smaller than the other comparative models, and the IDBO-SA-LSTM algorithm can predict the rolling force within ±4% with the hit rate of 99%, with high model accuracy and good generalization ability.
Lu Zhi , Liu Yinghuang , Zhang Xukun , Hou Rui
2025, 48(8):34-45.
Abstract:To solve the problems of low search efficiency, interspersed obstacles, unsmooth paths, and inability to avoid unknown obstacles in the traditional A* algorithm during path planning, a mobile robot dynamic obstacle avoidance method integrating the improved A* algorithm with the improved DWA algorithm is proposed. In the improved A* algorithm, the global obstacle occupancy ratio is introduced, dynamic weight coefficients are added to the heuristic function, the search field is optimized, the safe distance is set to remove redundant nodes, and third-order Bessel curves are added to smooth the paths, and the cost subfunctions of the target points are added to the DWA algorithm and the coefficients of the cost function are dynamically adjusted. The dynamic obstacle avoidance of the mobile robot is realized. Simulation results show that in different environments, compared with the traditional A* algorithm and other improved A* algorithms, the path length of the improved A* algorithm in this paper is shortened by an average of 5.14% and 1.01%, the search nodes are reduced by 57.05% and 36.59%, and the planning time is reduced by 34.39% and 8.49%, respectively; the improved fusion algorithm in this paper is reduced by an average of 5.14% and 1.01%, the search node is reduced by 57.05% and 36.59%, and the planning time is reduced by 34.39% and 8.49%, respectively. other fusion algorithms, the path length is shortened by 19.89% and 1.82% on average, and the planning time is reduced by 53.66% and 13.01% on average, respectively. It is proved that the improved fusion algorithm proposed in this paper effectively shortens the planned path length and time, and is able to realize real-time obstacle avoidance in complex dynamic environments to satisfy the high efficiency and safety of mobile robots during traveling.
2025, 48(8):46-54.
Abstract:Aiming at the problems of existing methods in dense, occluded and small object detection, this paper proposes a QARep-YOLOv8n algorithm for vehicle detection in urban road scenes. First, this paper adopts a Haar wavelet downsampling module to alleviate the problem of feature information loss caused by traditional stepwise convolution or pooling; secondly, this paper proposes a batch-normalization attention module and QARepC2f module to improve the feature extraction ability of YOLOv8; finally, this paper uses NWD bounding box loss and Slide classification loss to improve the detection effect of small and occluded objects. Extensive ablation experiments and validation experiments on four mainstream vehicle detection benchmark datasets shows that QARep-YOLOv8n improves mAP by 3.3%, 3.2%, 2.7% and 1.5%, respectively, compared with YOLOv8n. In addition, the proposed method has more significant detection effects on small and occluded objects.
Wang Chaohu , Lu Hongyi , Wu Wenyong , Li Linwei , Xiong Shuang
2025, 48(8):55-70.
Abstract:In order to solve the problems of unstable number of feature points, false detection, missed detection, poor size invariance, and low stitching accuracy under the traditional ORB algorithm in the leaf shape image stitching of aero engines, this paper carries out experimental research on leaf shape image stitching and proposes an improved corner detection ORB-CNN algorithm combined with a convolutional neural network. The idea of the algorithm is as follows: The improved corner detection algorithm is applied to realise corner extraction at different scales under the construction of the image pyramid. In the feature point detection stage, the convolutional neural network(CNN) is used to replace the FAST-n detection established by the 16-pixel ring in the FAST algorithm, and the convolutional layer is added to the CNN according to the number of pixels in the domain, that is, the design convolutional kernel is added to extract features related to FAST-n detection in the image. The BRIEF method was used to obtain the descriptor of the detected feature points, and the Hamming distance was calculated, so as to achieve accurate matching of the feature points. Experimental results show that compared with the traditional ORB algorithm and SIFT algorithm, the uniformity of feature point extraction of the improved algorithm is increased by 18.83% and 33.36%, respectively. In the illumination change experiment, the accuracy of feature point matching of the improved algorithm under strong light and dark light conditions is improved by 16.63% and 19.04%, respectively. Compared with the original ORB algorithm and SIFT algorithm, the feature point offset and matching accuracy are increased by 66.95%, 64.26%, 12.63% and 6.62%, respectively. This method effectively overcomes the limitations of the traditional ORB algorithm in terms of size invariance, and also retains the advantages of the ORB algorithm in terms of registration speed and quality, significantly improving detection performance and robustness in complex environments, and laying a foundation for the non-contact measurement of the blade gap of aero engines.
Guo Zhijun , Du Linlin , Wang Dingjian , Wang Yuan , Su Hao
2025, 48(8):71-79.
Abstract:To address the issues of path redundancy and low coverage in intelligent cleaning robot path planning, a hybrid algorithm for full coverage path planning is proposed, which integrates the semi-spring artificial potential field method, A* algorithm, and dynamic update strategy. This aims to solve the problem effectively. The A* algorithm is used for initial path planning, while the semi-spring artificial potential field method is employed for local obstacle avoidance to reduce local optima issues. The dynamic coverage value update strategy optimizes path priority based on real-time coverage, improving coverage efficiency and reducing redundant coverage. Additionally, the dynamic weight adjustment mechanism based on fuzzy logic enables the algorithm to adaptively adjust coverage and obstacle avoidance weights in complex environments. Simulation results indicate that the hybrid algorithm achieves higher coverage rates and lower redundant coverage compared to other algorithms. This has been further validated through real-world vehicle tests, demonstrating its capability to meet the full coverage operation requirements of cleaning robots.
2025, 48(8):80-87.
Abstract:Aiming at the throughput maximization problem of D2D-NOMA system under the condition of imperfect successive interference cancellation, a D2D-NOMA system throughput maximization algorithm based on imperfect SIC is proposed. Firstly, under the constraints of service quality and maximum transmission power of cellular users and D2D users, an optimization model with the goal of maximizing the throughput of D2D-NOMA system is established by considering the imperfect SIC condition. Then the model is converted into a Markov decision process with throughput as reward. A power allocation algorithm is designed using the TD3 algorithm to maximize the system throughput. The simulation results show that compared with the DDPG algorithm, the genetic algorithm and the random power allocation algorithm, the proposed algorithm improves the throughput of D2D-NOMA system under imperfect SIC by 14%, 25% and 40%, respectively, and also shows better convergence and fairness.
Cheng Jun , Mao Wei , Wang Buyun , Xu Dezhang , Yang Qiusheng
2025, 48(8):88-98.
Abstract:Stacking unmanned forklifts are responsible for the stacking and picking of goods in warehousing and logistics management. In industrial environments, it is necessary to develop fast and accurate state estimation and environment perception algorithms in order to achieve autonomous navigation movement of unmanned forklift trucks. However, when using LiDAR odometer for state estimation, problems such as inaccurate mapping and pose drift are often encountered. Therefore, a method of simultaneous localization and mapping of pileup unmanned forklift based on improved DLO algorithm is proposed. Firstly, the motion model provided by the inertial measurement unit and the point cloud data of the multi-line LiDAR are combined to perform a prior estimation of the initial pose of the forklift. Then, through the front end of DLO SLAM, the generalized least square method is used to scan and match, and the pose of the forklift is estimated in real time and the map is constructed. Finally, the back-end pose optimization and loop detection of HDL-Graph-SLAM are used to further improve the accuracy of map reconstruction. Experimental results show that the proposed scheme can effectively suppress map drift and error accumulation in dynamic environment. Compared with DLO SLAM, the localization accuracy is improved by 60.9% and compared with Cartographer algorithm by 56.9%. At the same time, the stability is also significantly improved to meet the requirements of stacking unmanned forklifts for accurate and efficient simultaneous localization and mapping.
Liu Pu , Ruan Zikang , Zhang Jingbo , Zhang Erliang
2025, 48(8):99-104.
Abstract:The estimation of the relative acoustic transfer function is a prerequisite for implementing multi-reference non-synchronous measurements with a microphone array. In industrial test scenarios, strong background noise poses a significant challenge, resulting in substantial errors in multi-reference non-synchronous measurements. To address such issue, an identification approach for the relative acoustic transfer function matrix based on total least squares is established. The cross-spectral matrix obtained by scanning the microphone array is completed, and acoustic holography imaging is achieved using the partial field derived from the decomposition of the spectral correlation matrix. The results indicate that the proposed method can effectively mitigate the influence of background noise on the completion of the spectral correlation matrix of microphone measurements under low signal-to-noise ratio scenarios. When the signal-to-noise ratio is below 10 dB, the error can be reduced by more than 2%, thereby enhancing the non-synchronous imaging accuracy of the microphone array.
Zhang Hao , Wang Hairu , Ma Jidong
2025, 48(8):105-115.
Abstract:To address the challenges of sparse labels and insufficient data in mechanical fault diagnosis while enhancing diagnostic performance and model interpretability, this paper introduces a time-frequency convolutional neural network(TF-CNN) model embedded with time-frequency analysis. By integrating advanced data augmentation techniques with a convolutional architecture leveraging time-frequency transformation, the model effectively extracts multi-scale, key time-frequency features from vibration signals. The time-frequency convolutional layer combines the physical interpretability of time-frequency analysis with the autonomous feature extraction capabilities of convolutional neural networks, enabling precise identification of critical signal characteristics and fault diagnosis through classification mechanisms. Experimental validation on the CWRU dataset demonstrates that the TF-CNN model achieves a diagnostic accuracy of 99.8%, significantly outperforming baseline methods. Additionally, frequency response analysis confirms the model′s ability to emphasize key signal frequency bands, further strengthening its physical interpretability. By seamlessly integrating the strengths of time-frequency analysis and deep learning, the TF-CNN model offers an innovative, efficient, and interpretable approach to industrial fault diagnosis. This work provides valuable insights and practical guidance for advancing fault diagnosis techniques, paving the way for robust applications in complex industrial scenarios.
Li Huipeng , Xu Bo , Huang Pu
2025, 48(8):116-125.
Abstract:To address the problem that the performance of the variational mode decomposition(VMD) algorithm for multi-component non-stationary mechanical vibration signals is severely affected by key factors such as the number of modes, the quadratic penalty parameter, and the update step size, a self-adaptive VMD algorithm based on a binary tree model is proposed. The quadratic penalty parameter is set based on the weighted fine-scale inverse scattering entropy of the decomposed signal, and the signal-to-noise ratio is used as a reference for the update step size. The original signal is continuously decomposed using the binary search mechanism. The optimal quadratic penalty parameter and update step size are continuously optimized, and the minimum least-squares mutual information and reconstruction error between the extracted components are used as the evaluation index for the decomposition completion. Modal merging is performed for modal features with high similarity. The algorithm comprehensively considers the common influence of embedded parameters on modal extraction performance. The proposed algorithm has low computational complexity and can completely adaptively extract the modal components of non-stationary signals, effectively alleviating the problem of overlapping bands between modalities with similar frequencies. The algorithm is validated by simulation data and real-measured mechanical vibration signals, and the experimental results show that the proposed algorithm has low computational complexity, can completely adaptively extract the modal components of non-stationary signals, and effectively alleviates the problem of overlapping bands between modalities with similar frequencies.
Yan Shuo , Wu Yang , Wang Shifeng , Wang Huiquan , He Jia
2025, 48(8):126-132.
Abstract:At present, since the method of measuring blood pressure with cuff cannot work in a high-pressure environment, in order to solve the problem of measuring blood pressure in a high-pressure environment, we propose a K-nearest neighbor continuous non-invasive blood pressure prediction model combining pulse wave conduction time and heart rate variability. In this study, rabbits were used for the experiment. They were pressurized from normal pressure to a depth of 1 000 m in an animal hyperbaric chamber. During this process, the electrocardiogram, pulse wave and invasive blood pressure of the rabbits were collected. Taking a duration of 30 s as one data point, and finally using data with a depth of 0~300 m for training and data with a depth of 300~1 000 m for prediction, the results of the mean absolute error±standard deviation of the K-nearest neighbor model in predicting the systolic and diastolic blood pressures of rabbit 1 were 2.2± 1.5 mmHg and 1.9±1.4 mmHg, respectively. The results of systolic and diastolic blood pressure for rabbit 2 were 1.7±1.3 mmHg and 1.7±1.5 mmHg, respectively. The results show that the method proposed in this paper has achieved good results in predicting blood pressure for different individuals in a high-pressure environment and provides ideas for blood pressure monitoring under high pressure.
Zhang Yu , Liu Haoyang , Jia Shengkai , Weng Qian , Zhang Heshan
2025, 48(8):133-143.
Abstract:To address the issues of large parameter and computation requirements, low detection accuracy, and the difficulty of efficiently deploying large models in safety helmet and reflective vest detection, an improved lightweight detection algorithm, CCEI-YOLOv8, is proposed. In this algorithm, the C2f-CIB module is adopted in the backbone and neck networks; the neck network is reconstructed with a cross-scale feature fusion module(CCFM); the EMA coordinate attention mechanism is introduced; and CIoU is replaced with Inner-EIoU to enhance regression localization accuracy. The effectiveness of the proposed algorithm is demonstrated through experiments conducted on the open-source Roboflow dataset for safety helmets and reflective vests. The results show that the algorithm achieves significant improvements: Parameters are reduced by 48.3%; computation is decreased by 32.1%; and the mean Average Precision(mAP@50) is increased by 0.5%, reaching 91.7%. The model size is reduced to only 3.442 MB, a decrease of 45%. Compared to the original YOLOv8n and other mainstream detection algorithms, CCEI-YOLOv8 demonstrates superior detection accuracy and lightweight design. This makes it highly suitable for real-time detection and deployment, providing a valuable reference for the real-time detection of safety helmets and reflective vests.
2025, 48(8):144-153.
Abstract:Bias correction is crucial for optimizing the prediction accuracy of traditional numerical weather prediction models. In meteorology, temperature is a vital parameter that significantly influences weather phenomena, atmospheric circulation, climate patterns, and human activities. Therefore, it is essential to perform temperature bias correction on traditional numerical weather prediction products. This paper designs a novel deep learning network model, SWGU-ConvLSTM, for temperature bias correction, which adopts the U-Net and bidirectional adversarial network architectures. The model uses the ConvLSTM module to extract local information and the SwinTransform module to capture global features. The outputs of the ConvLSTM and SwinTransform modules are then fused using the IAFF module. Furthermore, the fused feature information is processed with U-shaped connections and skip connections to better combine shallow and deep features and capture multi-scale information. Finally, the fusion module is employed as both the generator and discriminator in a bidirectional adversarial training framework to enhance the model′s learning and prediction capabilities. In this study, the TIGGE numerical model data from the ECMWF platform is used as the input data to be corrected, and ERA5 reanalysis data is used as the target data. The model is applied to correct 6-hour temperature forecasts. Experimental results show that the proposed SWGU-ConvLSTM model outperforms other comparison models in terms of MSE, MAE, and SSIM. Compared to the simvp model, its MSE and MAE errors are reduced by 30% and 27%, respectively, improving the accuracy of temperature bias correction.
Li Zuopeng , Liu Jiaxiang , Zhang Shaowen
2025, 48(8):154-164.
Abstract:Aiming at the current problems of occlusion, noise interference and low detection accuracy between prohibited X-ray objects, a contraband detection model integrating anchor-aided training strategy and fine-grained multi-scale features was proposed based on the YOLOv8s network. In the network, the C3_Res2Net module is used to replace the C2f module. By integrating features at different levels to enhance multi-scale, the receptive field range of the network layer is increased, and features at the fine-grained level are obtained to solve the problem of low detection accuracy caused by occlusion between contraband items;the sliding average Slide Loss target category loss function and the improved border loss function are used to try to assign higher weights to difficult samples, which reduces the competitiveness of high-quality anchor frames while reducing the harmful gradients generated by low-quality examples. At the same time, the focus is on anchor frames of ordinary quality to improve the overall performance of the detector and make it have better anti-noise interference ability; in the early stages of training, the ATSS(Adaptive Training Sample Selection) and Task-Aligned Assigner collaborative label assignment strategies are used, leveraging anchor-based preset information to stabilize model training; in the later training stages, an anchor-aided training strategy further enhances detection accuracy by exploiting the respective advantages of various anchor networks; the improved model was trained and tested on the public SIXray and HiXray datasets, achieving mAP50 scores of 94.9% and 83.7%, and mAP50:95 scores of 73.1% and 52.2%, respectively. The results demonstrate that the proposed model offers high accuracy and stability in contraband detection.
2025, 48(8):165-176.
Abstract:To tackle challenges such as complex backgrounds, scale variability, and difficulty in detecting small objects in X-ray security inspection images, a lightweight contraband detection algorithm named LEM-YOLO is proposed, focusing on edge and multi-scale features. Firstly, a lightweight edge feature enhancement (LEFE) module is designed to strengthen edge feature extraction. Secondly, an efficient multi-level feature fusion pyramid network is developed, incorporating dynamic upsampling (Dysample) and the hierarchical scale feature pyramid network (HS-FPN) to enhance multi-scale feature fusion while reducing computational redundancy. Additionally, a dynamic feature encoding (DFE) module is used to preserve global information for small objects. Finally, Shape-IoU is employed as the bounding box regression loss function, concentrating on boundary shape and scale to improve localization accuracy. Experimental results on the public SIXray dataset demonstrate that LEM-YOLO achieves a mAP of 94.63%, a 2.56% increase over the original algorithm, while reducing model size by 50.67%, making it more suitable for contraband detection scenarios.
Xiao Hengshu , Li Junying , Liang Hong , Ma Erdeng , Zhang Hong
2025, 48(8):177-186.
Abstract:The estimation of tobacco leaf yield is a crucial task, as the number of leaves directly impacts the yield. Traditional manual statistics are inefficient and costly, in order to solve these problems, this research proposes a lightweight YOLOv8-SLSS tobacco leaf counting detection algorithm, which improves on the YOLOv8n methods for the lack of detection accuracy, high computational complexity, and missed detections caused by overlapping tobacco leaves. The algorithm replaces the original model′s backbone network with an enhanced ShuffleNetV2light architecture, reducing model parameters and computational load. Integrate the LHCB module into the neck network′s C2f module to expand the model′s receptive field, enhances detection capabilities and reduces computational load. The introduction of the SEAMDetect module has enhanced the detection capabilities in scenarios involving occlusion by tobacco leaves. Finally, the SPPELAN module is introduced to enhance the model multi-scale feature extraction capability and computational efficiency. Experimental results demonstrate that the modified model significantly reduces model parameters and floating-point operations by 63.3% and 61.7% respectively. The algorithm′s average precision improves from 91.8% to 93.1%, achieving a real-time detection speed of 83 fps, marking a 51% enhancement over the original algorithm, meeting real-time detection demands. The improved algorithm enhances the detection ability of the traditional YOLO model in tobacco leaf occlusion scenarios, realizes the balance of high accuracy, lightweight design, and real-time detection performance, thus providing effective technical support for the digitization of tobacco agriculture.
Sun Haiqing , Yang Chuanying , Ao Legen
2025, 48(8):187-195.
Abstract:In order to reduce the interference of environmental factors such as complex weather, lighting changes, and traffic sign fouling, as well as the degradation of model performance caused by the high complexity of the algorithm itself, a highway road traffic sign detection algorithm based on RT-DETR as the benchmark model is proposed. Firstly, the designed lightweight module CSP-PMSFA is used as the backbone network of the algorithm to reduce the computational and parameter complexity of the model and improve its expressive power. Then, to address the issues of high computational complexity, small model capacity, and limited computational efficiency in the feature interaction module within the original algorithm scale, cascaded group attention CGA was introduced for improvement. Finally, a cross scale feature fusion module EMBSFPN was designed to address the issues of insufficient receptive field adaptability and limited feature information processing; the decoding mechanism using EUCB upsampling module preserves and fuses feature information while ensuring accuracy, optimizing performance and improving the robustness of the model. The experimental results show that the improved algorithm has improved mAP50 by 2.6% and FPS by 13.6 frames compared to the original algorithm on the ROAD MARK road marking detection dataset. The computational and parameter requirements have decreased by 29.7% and 39.6%, respectively. Overall, it outperforms other improved algorithms and improves detection accuracy and speed while being lightweight, demonstrating practicality.
Editor in chief:Prof. Sun Shenghe
Inauguration:1980
ISSN:1002-7300
CN:11-2175/TN
Domestic postal code:2-369