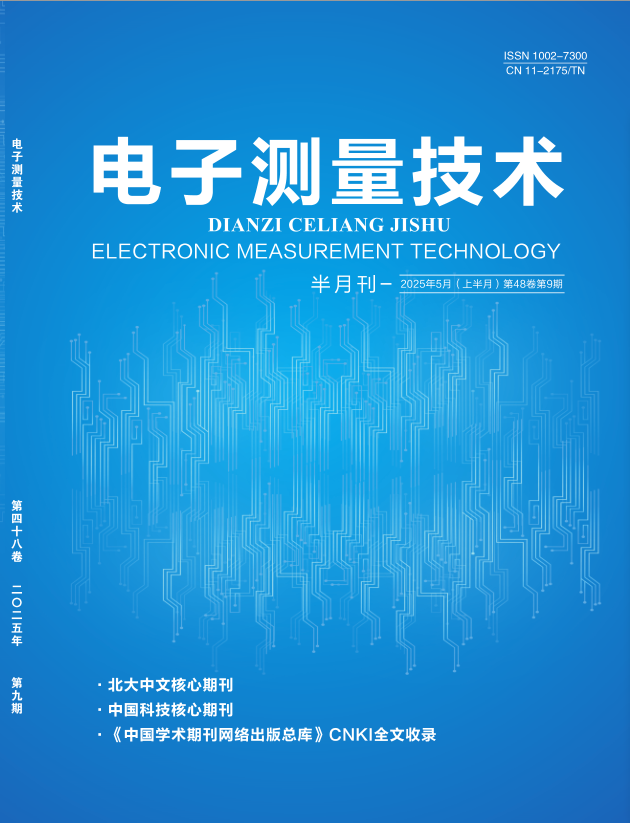
Editor in chief:Prof. Sun Shenghe
Inauguration:1980
ISSN:1002-7300
CN:11-2175/TN
Domestic postal code:2-369
- Most Read
- Most Cited
- Most Downloaded
Zhou Xingwei , Hu Wenshan , Liu Guoping , Lei Zhongcheng
2025, 48(5):1-12.
Abstract:As modern control systems demand increasingly higher real-time performance and precision, microsecond-level sampling real-time control technology has become a research hotspot. To address the reliance on foreign platforms in existing solutions, this paper presents a microsecond-level sampling real-time control system based on the STM32 microcontroller, designed with independent intellectual property rights. The system addresses key challenges, including the real-time performance of control tasks, the efficiency of communication tasks, and the complexity of automatic code generation. It employs the FreeRTOS real-time operating system for task scheduling, hardware timers for high-priority execution of control tasks, and the LwIP protocol for low-latency network communication, enabling efficient data uploading and command reception. Furthermore, the paper proposes a graphical block diagram-based approach for control algorithm parsing and automatic code generation, where a server parses user-designed block diagrams and generates optimized control code tailored for STM32 hardware. Experimental validation using the field-oriented control of a permanent magnet synchronous motor demonstrates the system′s excellent real-time performance and control capability under microsecond-level sampling. This system effectively overcomes the constraints imposed by foreign technological barriers, significantly enhancing the development capabilities of domestic control systems. The proposed solution offers a viable approach to addressing critical “bottleneck” issues in key technologies and holds great potential for wide-ranging applications and scalability.
Yang Kang , Xing Hongyan , Wang Zhoulong
2025, 48(5):13-21.
Abstract:In view of the problem that the lightning electromagnetic pulse invades the transmission channel of the communication system, resulting in interference or even damage to the power amplifier, a lightning protection module is designed to realize the decrease of the amplitude and specific frequency components of the lightning electromagnetic pulse, and protect the power amplifier from the influence of lightning intrusion. The module comprises a transient protection circuit and a filtering circuit, and the transient protection circuit is mainly to limit the lightning-induced voltage and transfer the relevant current caused by lightning; the filter circuit suppresses the low-frequency components of lightning below 1 MHz. After simplifying the transmission channel of the communication system, installing the protection module and simulating the lightning current intrusion of 8/20 μs, the peak power of the power amplifier will not exceed 30 dBm, which is less than the minimum value of the tolerable peak threshold power range. The experiment carries out the intrusion of lightning electromagnetic pulse on the simplified transmission channel, and the gain data shows that there is no obvious gain compression of the amplifier at the center frequency of 144 MHz, which indicates that the protection module can effectively protect the sensitive components.
He Shaodong , Tang Changliang , Xu Jingwen , Song Jinhua , Mu Mengliang
2025, 48(5):22-29.
Abstract:In order to more effectively suppress the vibrations of the vertical rotor system, an electromagnetic damper based on active electromagnetic force is proposed as a replacement for the traditional oil film damper, which relies on viscous damping force for vibration reduction. The magnetic circuit structure is simulated and validated using finite element analysis. Based on the dynamic model of the damper under the influence of electromagnetic force, a corresponding PD control system is designed. A vertical rotor acceleration test rig was set up, in which both the electromagnetic damper and the oil film damper were employed as the support structures for the system, respectively, to perform vibration suppression tests and analysis. The experimental results demonstrate that the support characteristics of the electromagnetic damper are dynamically adjustable and exhibit excellent vibration suppression performance with appropriate control parameters, showing a 46.49% reduction in amplitude compared to the oil film damper. Moreover, after integrating a phase lead compensator into the PD controller, the rotor's amplitude in the critical region decreased by 15.78%, while the low-frequency vibration energy was reduced by 86%. The research findings validate the feasibility and applicability of the electromagnetic damper for vibration suppression in vertical rotor systems, while also identifying directions for future optimization.
Yang Yong , Hu Dong , Dai Hao , Tang Chao , Xie Jufang
2025, 48(5):30-39.
Abstract:In response to the fact that the accuracy of traditional transformer intelligent diagnosis models based on dissolved gas analysis (DGA) data is easily affected by the selection of input feature dimensions and hyperparameters, this study proposes a transformer intelligent fault diagnosis model based on the combination of real domain rough sets and NRBO-XGBoost. Firstly, a feature extraction algorithm with adaptive performance is proposed based on the concept of real domain rough set for extracting initial fault features of transformers; secondly, in response to the limitation of XGBoost being affected by hyperparameter selection in transformer fault diagnosis, this study utilizes the high convergence speed and effective avoidance of local optima of the NRBO algorithm to globally optimize the hyperparameters of XGBoost, and proposes the NRBO-XGBoost model for transformer fault diagnosis; finally, through multiple experimental comparisons, compared with other traditional features, the performance of the feature extraction algorithm proposed in this study has been improved in various classifiers, proving that the feature extraction algorithm proposed in this paper can effectively extract information from the features to enhance the performance of the model. Moreover, NRBO-XGBoost achieves an accuracy of 92.09% in transformer fault diagnosis with only 20 convergences compared to other comparative models, demonstrating superior performance.
Zhou Xiang , Wang Keqing , Zhou Xinxiang , Han Jitai
2025, 48(5):40-49.
Abstract:For the electric vehicle helmet wearing detection method exists in complex road conditions helmet small target detection accuracy is low, the target mutual occlusion leakage rate is high, detection model large arithmetic complexity and other problems. This question proposes a target detection algorithm based on improved YOLOv10n to solve these problems in practical applications. Firstly, the advantages of BiFPN are integrated on the basis of MAFPN, and the BIMAFPN structure is innovatively proposed, which improves the detection performance of the model in complex road scenarios. Secondly, the Inner-Wise-MPDIoU loss function is constructed to replace the traditional CIoU loss function, which improves the detection accuracy of the network while accelerating the convergence process of the model. Finally, the LSCD detection head is introduced to further reduce the number of model parameters and improve the detection performance. Experimental results show that compared with the original model, the improved model improves the accuracy of mAP@0.5 by 2.7%, the number of parameters is reduced by 25%, and the model size is reduced by 35%. The detection method used in this paper not only significantly improves the accuracy of helmet detection under complex road conditions, but also maintains good real-time performance while taking into account lightweight, which makes it easy to deploy the model in small embedded transport devices.
Wang Jinnan , Zhang Xiaoming , Zhang Ge , Zhao Chenyang
2025, 48(5):50-56.
Abstract:In order to solve the problem of magnetic interference caused by airborne equipment and motion characteristics in UAV magnetic anti-submarine missions, a study based on the improved magnetic compensation model was carried out. An improved T-L magnetic compensation model based on GAN and LSTM is proposed, which combines the advantages of the traditional T-L model in attitude-dependent noise modeling, the ability of LSTM to capture the long-term interval dependence, and the learning ability of GAN to the data distribution characteristics, so as to significantly improve the accuracy of noise recognition and the measurement accuracy of magnetic data. The simulation results show that the compensation accuracy is increased by 67% and the improvement ratio is 43.67 compared with the T-L model, and the compensation accuracy is increased by 64% and the improvement ratio is 26.93 in the UAV airborne test. The results show that this method significantly improves the quality of magnetic survey data and provides accurate magnetic field for underwater anti-submarine missions based on magnetic field changes.
Xiao Xia , Ma Qiang , Yang Zhen
2025, 48(5):57-64.
Abstract:Aiming to address the problems of randomness and low prediction accuracy in electric vehicle charging load forecasting, the paper proposed a novel approach based on TCN. This approach integrated variational mode decomposition, attention mechanisms and multi-scale features, leading to the development of the electric vehicle load forecasting model, VMD-AM-MSF-TCNnet. Firstly, the proposed method utilizes variational mode decomposition, optimized using the whale optimization algorithm, to decompose the electric vehicle charging load sequence. Secondly, the method introduced a gating mechanism and dual attention mechanisms to enhance the residual blocks of the original TCN. The model achieved multi-scale feature fusion by combining the attention-enhanced outputs of improved TCN residual blocks of varying sizes. Finally, it finalized the prediction through the reconstruction of the load components. The experimental results indicate that the proposed model demonstrates improvements in the performance metrics of RSE、 RAE and R2 compared to the original TCN, showing that it has a good predictive performance.
Xin Fengyue , Kou Zhiwei , Liu Yanchen , Cui Xiaoming , Qi Yongsheng
2025, 48(5):65-73.
Abstract:Cloud cover can cause dramatic fluctuations in photovoltaic (PV) power, which poses a great challenge to the sTable operation of the power system. In order to address the problems of large fluctuation of PV power with cloud cover and low accuracy under highly complex weather conditions, we propose an ultra-short-term PV power prediction model based on two-stream feature enhancement network (TSFE-LSTM). Firstly, the groundbased cloud images are processed by fish-eye image correction algorithm and optical flow estimation algorithm, and the spatiotemporal feature of the ground-based cloud image is extracted by the two-stream convolutional network. Secondly, an ultra-short-term PV power prediction model based on two-stream feature enhancement network is built to predict the PV power in the next 15 min. The results of the experiment show that the MAE and RMSE of the TSFE-LSTM model for PV power prediction in the next 15 min are 6.49% and 10.76% with the input of ground-based cloud image and optical flow, respectively, which are improved by 5.81% and 4.61%, respectively, compared with the single input data. In sunny conditions, the MAE and RMSE of TSFE-LSTM model improved 7.23% and 14.99% than the CNN model, respectively. In cloudy conditions, the accuracy of the model is slightly de-creased, but the MAE and RMSE are improved by 3.53% and 1.26%, respectively, compared with the CNN-MLP model. This provides new ideas for PV power prediction with cloud cover.
Chen Shuaiyin , Liu Ning , Hu Kejun
2025, 48(5):74-80.
Abstract:In order to solve the problems of low positioning accuracy and poor continuity in the single navigation source positioning system in indoor and outdoor seamless positioning, a GNSS/UWB/IMU integrated indoor and outdoor seamless navigation and positioning algorithm based on robust estimation was proposed. In the face of complex indoor and outdoor scene switching, the robustness estimation algorithm is used to evaluate the confidence level of the two observation signals collected by GNSS and UWB and fuse them, and the fused data is used as the new observation value, and the extended Kalman filter algorithm is used to fuse the new observation value with the data of the inertial system to achieve fusion positioning. In order to evaluate the navigation and positioning accuracy of the algorithm in the presence of interference and noise, the inertial navigation positioning module, the satellite positioning module and the ultra-wideband tag were integrated together and field tests were carried out. Experiments show that the root mean square error of the proposed fusion positioning method is 6.40 cm in the east direction and 6.73 cm in the north direction, and the maximum error is not more than 28.55 cm.
2025, 48(5):81-91.
Abstract:An improved algorithm based on the YOLOv8n model is proposed to address the challenge of detection accuracy for electric vehicles entering elevators in complex scenarios.First, the backbone network integrates the C2f module with the universal inverted bottleneck structure to form a new C2f_UIB module, optimizing computational efficiency and reducing parameters while improving global information capture capability. Additionally, a spatial-channel synergistic attention (SCSA) module is added to the backbone to enhance feature extraction and model robustness. Second, the neck network is reconstructed using an improved re-parameterized generalized feature pyramid network (DSRepGFPN), which enhances cross-scale feature fusion, improves multi-scale object detection performance, and reduces computational complexity. Finally, the original CIOU loss function is replaced with MPDIOU, improving bounding box localization accuracy, especially in scenarios with lighting variations and object occlusions. Experimental results on an elevator electric vehicle dataset show that the proposed YOLOv8-UAR algorithm achieves a 2.5% improvement in mAP50 and a 1.8% improvement in mAP50.95 compared to YOLOv8n, with a detection speed of 94 fps. This makes it suiTable for deployment on edge devices, aligning better with practical requirements for electric vehicle elevator detection.
Yan Huabiao , Li Dongli , Huang Lyu′e , Zhang Hangsong , Yao Longlong
2025, 48(5):92-101.
Abstract:In order to solve the problem that the time series features cannot be fully extracted due to the characteristics of power load, such as nonlinearity, high volatility and strong randomness, a short-term power load prediction method based on improved complete ensemble empirical mode decomposition with adaptive noise and a deep error correction multi-scale bidirectional temporal convolution network-bidirectional long short-term memory network is proposed. First, the maximum information coefficient is used to select a highly relevant feature set to reduce feature redundancy. Then, improved complete ensemble empirical mode decomposition with adaptive noise is employed to decompose the highly fluctuating load into intrinsic mode functions with different frequencies and residual, while sample entropy is introduced to reconstruct new subsequences with similar complexities to reduce computational load. Next, using the optimal feature set, each subsequence is forecasted with a multi-scale bidirectional temporal convolution network-bidirectional long short-term memory network model optimized by the sparrow search algorithm to obtain initial load sequence predictions. Finally, the error sequence is corrected using the error correction module to refine the initial predictions. Experimental verification shows that compared with other prediction models, RMSE is reduced by 51.42% at most and 34.26% at least, which verifies the accuracy and effectiveness of the model.
2025, 48(5):102-110.
Abstract:In this study, a ovel deep learning model named ShuffleVT was proposed to estimate continuous finger force base on surface electromyography (sEMG). This model was composed of the basic units of ShuffleNetV2 and the Vision Transformer (ViT) structure. Its performance was validated on a publicly available NinaPro database, which includes sEMG data from 40 healthy subjects and finger force data from 6 degrees of freedom. Three performance metrics including Pearson correlation coefficient (CC), root mean square error (RMSE), and coefficient of determination (R2) were used. And another four deep learning models, ShuffleNetV2, ViT, Transformer, and LSTM were included for comparison. The results showed the averaged CC, RMSE, and R2 was 0.92±0.05, 1.27±0.66, and 0.83±0.10, respectively, significantly better than that computed with another four models. It indicates that the newly proposed ShuffleVT model could by potentially applied into the sEMG-driven continuous estimation of human motor intention.
Wei Zhengchao , Qiao Qiang , Lang Junjie , Yang Hongjie
2025, 48(5):111-117.
Abstract:For the problem that closed set networks cannot effectively identify unknown classes in open set scenarios, an open set specific emitter identification(SEI )method based on the combination of deep learning and unsupervised clustering was proposed. The original data is refined to extract the conventional features containing fingerprint features, and the conventional feature waveform is trained in the closed set network,based on probability measures, the known and unknown decisions are carried out on the identification class samples, and unsupervised dimension reduction and clustering are carried out on the features of the full connection layer of the residual network extracted from the samples identified as unknown classes. The number of unknown individuals was clarified the clustering results. The experimental results show that compared with traditional closed set recognition, the proposed method can realize the effective classification between known classes and unknown classes ,under the known classes accurate recognition, the recognition accuracy is more than 97%, and ten unknown radios can be accurately clarified,experimental results verify the effectiveness of the method。
Song Jiayu , Gao Xuelian , Chen Zhexuan
2025, 48(5):118-127.
Abstract:To tackle the challenge of multiple concurrent fault localization in analog circuits, we propose a localization algorithm that integrates both real and complex domains. In the real domain, comprehensive data features are extracted by employing various operations of spatial and channel attention mechanisms, while simultaneously managing network depth and parameter amount. Within the complex domain, we capitalize on the disparities emerging across layers within a complex-valued convolutional neural network. Through skip connections, a deep-shallow feature fusion structure is formulated, ensuring that shallow information susceptible to loss is preserved and integrated with deep information to yield complex-domain features. The integration of real and complex domain features is subsequently applied to the research on composite fault localization in analog circuits, yielding an average localization accuracy exceeding 93% and a peak accuracy reaching 100%. This approach demonstrates robust stability and reliability, furnishing a viable solution for the ongoing research on composite fault localization in analog circuits.
Wang Wei , Yu Xin , Miu Jiaxin , Liu Xiaoyu
2025, 48(5):128-136.
Abstract:The appearance of defects on the surface of solar cells will seriously affect the efficiency of solar energy conversion, accurate detection of defects on the surface of solar cells and timely treatment can effectively improve power generation efficiency. Aiming at the demand for high precision and real-time surface defect detection in the solar cell production process, this paper proposes a solar cell surface defect detection algorithm based on the improved YOLOv5s. The algorithm firstly replaces the C3 module in the network with a C3CA module in the backbone feature extraction network, and incorporates the CBAM attention mechanism to improve the feature extraction capability of the network; secondly, introduces the BiFPN network structure in the feature fusion network to improve the feature fusion capability of different semantic and scale information in the network; lastly, introduces a decoupled head in the output end, which improves the convergence speed of the modeled network with the detection accuracy. The experimental results show that the improved model has an average precision of 66.4% on the EL dataset of photovoltaic cells with an mAP@0.5∶0.95, which is 7.1% higher than the original network. It realizes the fast and effective localization and identification of defects on the surface of solar cell wafers, which has certain practical application value in the industrial production process of solar cells.
Zhang Jianing , Han Fengxia , Wang Hongjun , Liu Shucong , Wang Jun
2025, 48(5):137-146.
Abstract:Common defects in 3D printed carbon fiber reinforced composite components include cracks, bubbles, delamination, and layering issues. However, traditional infrared thermography processing techniques face problems such as edge blurring and artifacts, and they do not fully utilize the temporal information of pixel values. Therefore, this paper proposes an adaptive median filtering algorithm based on temporal information. By combining changes in the current pixel over time and using the z-score outlier removal method, the algorithm assesses whether the current pixel is anomalous within a specific time frame, thereby reducing noise interference. Experimental results demonstrate that this algorithm achieves an average signal-to-noise ratio that is 6-155 dB higher than methods such as wavelet denoising and tensor principal component analysis, while maintaining good edge definition. Additionally, defects were quantified using the half-width measurement method, maximum inter-class variance method, and Gaussian Laplacian operator. The experiments indicate that the content of carbon fiber and the excitation time significantly affect the accuracy of defect quantification.
2025, 48(5):147-155.
Abstract:In order to solve the problems of single battery style, less feature information and limited academic scenario, we focus on lightweight detection algorithm, and propose an electric bicycle battery detection algorithm based on an improved version of YOLOv8, termed PSPG-YOLO. In terms of feature extraction within the network mesh, have designed a multi-branch PStarblock to optimize C2f module enhancing the featureinformation representational capacity with a simpler module. For multi-scale fusion feature pyramid, dilated convolution with shared parameters is employed to refine the SPPF structure, increasing receptive fields while preserving more detailed feature information. regarding detection head design, we introduce a GSPH. By leveraging shared convolution alongside partial convolution techniques, we reduce model complexity,additionally, both anchor point and stride can be dynamically adjusted; internal parameters are automatically calibrated to enhance adaptability across various scale feature maps. Experiments conducted on a self-constructed data-set specifically targeting electric bicycle batteries demonstrate that, compared to the baseline model YOLOv8n, PSPG-YOLO achieves reductions in FLOPs by 57% and in params by 43%,and improved mAP50 values by 0.8,yielding superior overall performance relative to other mainstream detection models,providing an efficient management method for electric bicycle battery illegal entry into households.
Miao Shaojie , Li Xian , Zhao Dongjie , Sun Yu
2025, 48(5):156-165.
Abstract:Visual servo control robots mainly rely on their vision systems to detect corner points, edges, and circles of workpieces, providing the basis for subsequent decision-making and control. With the rapid increase of its workload density, the edge modules struggle to meet these detection loads, especially for continuous batch detection in complex scenarios, where the real-time and accuracy of visual system are greatly challenged, reducing the system efficiency. To deal with the above bottlenecks in circle detection, particularly the more challenging circle detection, we proposed a novel synchronous circle detection method based on Hough gradient: the interference information in the image is removed by edge screening of the edge image; the center and radius are synchronously determined by the eight-point method; the radius error is reduced by radius re-search, and invalid calculations are reduced by center position constraints; the accurate detection of circular targets in the image is achieved by candidate circle re-search and optimal circle acquisition. To further enhance the speed and efficiency of circle detection, the above method is integrated with CUDA parallel technology for a synchronous parallel circle detection method based on Hough gradient, which fully utilizes its parallel computing to accelerate the circle detection. In comparison with GHT, CACD, RCD, and Zhao, the proposed method significantly improves the circle detection accuracy and efficiency with stronger anti-noise and anti-disturbance capabilities, where its precision, recall rate and F value are 99.1%, 90.7% and 94.7% respectively; the average detection time is 0.09 s per image with an efficiency increase by up to 26 times, making it suitable for batch image processing in industry.
Zeng Derong , Tan Gan , Zhong Minliang , Gao Weiwei , Lan Jieqin
2025, 48(5):166-174.
Abstract:Lithium batteries, as an important component of the current new energy market, are widely used in consumer electronics, electric vehicles, and energy storage fields. To ensure the safety of lithium batteries, they are usually inspected internally using X-rays before leaving the factory to eliminate defective products. However, the original images of lithium batteries using X-ray imaging often have low contrast, uneven contrast, and concentrated grayscale values in narrow areas, making it difficult to distinguish the detailed information of the sample. This article proposes two methods to enhance the contrast of lithium battery X-ray images based on the above characteristics. The first method is to use a custom designed nonlinear grayscale transformation function combined with the CLAHE algorithm to enhance contrast, the second method is to use homomorphic filtering combined with CLAHE transform to enhance contrast. This article developed a program to implement the idea and tested on cylindrical wound lithium battery images. The results showed that the contrast enhancement algorithm proposed in this article increased the information entropy of the image by about 20%, made the grayscale histogram display more uniform, has higher contrast ratio, and then the quality of lithium battery X-ray images improved significantly. This study will provide technical support for the micro focus X-ray defect detection and automatic recognition of lithium batteries.
Li Bo , Xu Ziwei , Zhong Fei , Chen Yihua
2025, 48(5):175-183.
Abstract:Aircraft landing gear defect detection is of great significance to ensure flight safety. Aiming at the problems of insufficient accuracy and large number of parameters in the existing target detection methods, an aircraft landing gear defect detection method named GSV-YOLO is proposed, which is based on YOLOv7-tiny and improved. Firstly, Ghost convolution is used to replace the standard convolution in the YOLOv7-tiny model to realize the model lightweight and reduce the number of parameters; secondly, a depth-separable self-attention module (SepViT) is introduced to enhance the model′s ability of extracting the global information and to reduce the misdetection and underdetection of small target samples; a lightweight detector head is designed to strengthen the model′s classification and localization ability; finally, the dynamic non-monotonic focusing mechanism based on Wise-IoU loss to focus on common quality anchor frames to further enhance the detection performance. The experimental results show that GSV-YOLO achieves an average detection accuracy of 80.4% on the aircraft landing gear defect dataset, which is 4.9% higher compared to the original model, and the amount of model parameters is reduced by 10.6%, which improves both the model scale and detection accuracy. Applying this method to industrial environments significantly improves the detection efficiency and has great application value.
Yang Li , Deng Jingwei , Duan Hailong , Yang Chenchen , Li Fengquan
2025, 48(5):184-192.
Abstract:A defect detection algorithm OM-Detector based on reparameterization was proposed to solve the problems of uneven background interference, variable shape and multi-scale defects in electroluminescence image of photovoltaic cells. Firstly, OREPANCSPELAN4 module is proposed by combining generalized high-efficiency layer aggregation network and online reparameterization. The introduction of heavy parameterization can effectively train through gradient descent optimization algorithm, which can improve the accuracy and reduce the number of model parameters, making the model lightweight. Secondly, a multi-scale convolutional attention module is introduced into the neck network to suppress the interference of complex background and improve the accuracy of the model to detect fine defects. Finally, a defect detector is constructed by combining the heavy parametric feature extraction-fusion module and the multi-scale convolution attention module. The performance of the algorithm was verified by using the photovoltaic cell anomaly detection data set. The experimental results showed that compared with the YOLOv8 detection network, the mean average precision was increased by 2.5%, the number of parameters was reduced by 29%, and the reasoning speed was accelerated by 5.7%, which was superior to the current mainstream target detection algorithm and could detect the surface defects of photovoltaic cells quickly and accurately.
Editor in chief:Prof. Sun Shenghe
Inauguration:1980
ISSN:1002-7300
CN:11-2175/TN
Domestic postal code:2-369