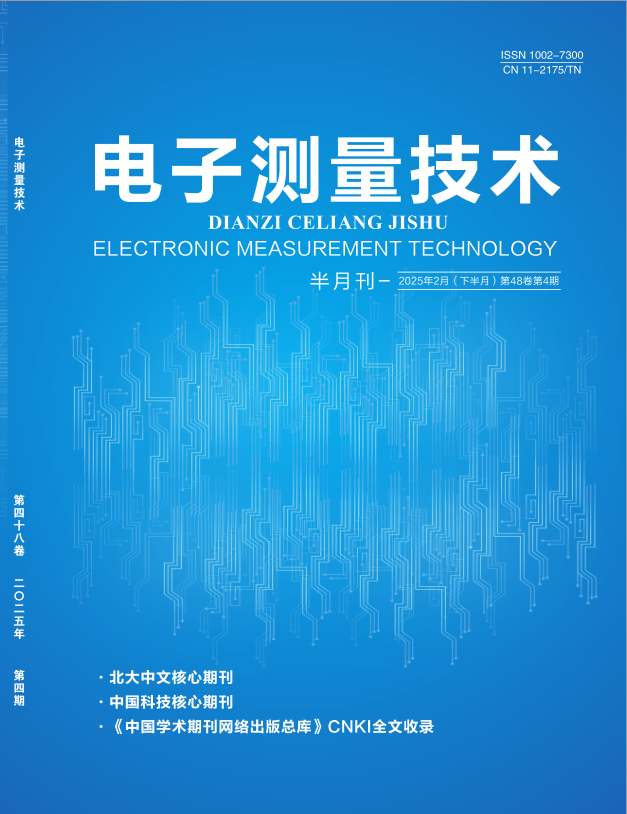
Editor in chief:Prof. Sun Shenghe
Inauguration:1980
ISSN:1002-7300
CN:11-2175/TN
Domestic postal code:2-369
- Most Read
- Most Cited
- Most Downloaded
Huang Wei , Ma Jiaqing , Chen Changsheng , He Zhiqin , Wu Qinmu
2025, 48(4):1-7.
Abstract:In order to improve the control performance of permanent magnet synchronous motor, a fuzzy backstepping control strategy of permanent magnet synchronous motor based on differential evolution algorithm was proposed to solve the problems of large overshoot and poor anti-interference performance of permanent magnet synchronous motor when changing speed under fuzzy PID control. Firstly, the fuzzy control strategy is combined with the backstepping control theory to adjust the structural parameters of the speed loop and the current loop. Then, on this basis, the differential evolution algorithm is used to optimize the structural parameters in the fuzzy controller. Finally, the proposed control strategy is verified by simulation and experiment. The experimental results show that the speed drop of the motor is reduced by 1.78% under the proposed control strategy. Under no-load conditions, the overshoot of speed is reduced by 22.9% when the speed is lowered and 3.5% when the speed is raised, which improves the anti-interference ability of the system.
Cheng Zhe , Liu Zutao , Wu Qiong
2025, 48(4):8-15.
Abstract:A high-efficiency isolated DC-DC converter based on a coreless micro-transformer was designed using the GSMC 0.35 μm BCD process. The effects of line width, inner diameter, line spacing, number of turns and distance between primary and secondary coils on the efficiency of isolated DC-DC converter are analyzed, and a set of coreless miniature transformer models with the best EMX modeling and simulation data are applied to the isolated DC-DC converter. Finally, the optimal coding and decoding scheme is selected. Further improve the conversion efficiency of the converter. Simulation results indicate that at a working frequency of 180 MHz, with an input supply voltage of 5 V and an output isolated voltage of 5 V, the converter can output a current range of 0 to 120 mA, with a maximum output power of 600 mW and a peak conversion efficiency of 40%.
Sun Dongdong , Chen Dan , Xu Zhezhuang , Zhang Hanlin
2025, 48(4):16-24.
Abstract:Aiming at the problems of low search efficiency of FMT* algorithm in robot arm path planning and easy planning failure when there are fewer sampling points, this paper proposes a US-FMT* algorithm based on uniform sampling to improve the planning success rate and search efficiency. The algorithm first adopts a uniform division of the global workspace to generate random sampling points, which improves the performance of the algorithm under low sampling point conditions; then, it is combined with the neighbor node search-oriented strategy to reduce redundant node exploration in the planning process; finally, it adopts the node reconnection strategy to optimize the quality of the path. The algorithms are experimentally analysed in 2D and 3D simulation environments, and path planning experiments are performed on an ABB IRB 1200-0.7/7 kg robotic arm. In the 3D path planning simulation environment, the US-FMT* algorithm reduces the time cost by about 49.7% and the path cost by about 16.6% compared to the FMT* algorithm, which shows that the US-FMT* algorithm is able to effectively improve the planning success rate of robotic arm path planning, provide excellent path quality and lower computational cost, and provide an efficient solution for robotic arm motion planning.
2025, 48(4):25-34.
Abstract:Aiming at the need of improving ride comfort and the limitation of relying on a single control algorithm, this paper presents a hybrid control method with simple structure, high performance and low application cost. Firstly, a variable index ceiling control is designed, and the simulation shows that the control effect is better in the low frequency band and better than the traditional ceiling. Secondly, the LQG control is designed, and the Q and R matrix is optimized by genetic particle swarm optimization algorithm to obtain the global optimal solution. According to the analysis, the control effect is better in the high frequency region and the algorithm is mature and stable. Then the two are fused by the way of high and low frequency division to realize the joint control of the electric controlled air suspension damping in the full frequency band. Finally, a simulation model of 1/4 car electronic control air suspension based on MATLAB/Simulink platform and a hardware-in-the-loop experiment platform (HIL) based on U2 rapid control prototype and dSPACE/Simulator real-time simulator are built. Simulation and experimental verification are carried out under random road conditions. Compared with passive suspension and SH-ADD hybrid control, the acceleration of the body decreases by 15.53% and 6.42%, respectively, which achieves better control effect in the whole frequency band and fully improves the ride comfort.
Luo Bin , Chang Jun , Sun Jiangli , Li Dong
2025, 48(4):35-43.
Abstract:Aiming at the problems of excessive computational complexity and large number of parameters in existing radar fall detection methods, this paper proposes a lightweight adaptive convolutional neural network, LACNN.Firstly, micro-Doppler features are extracted from the human activity echo signals acquired by the FMCW radar. Secondly, Initial extraction of features using an improved lightweight ShuffleNet network. Then, the features are refined using a lightweight channel-space attention module with an efficient asymmetric convolutional kernel parallel multi-scale feature extraction module. To improve the generalisation ability of the model, a convolutional batch normalization AconC module is embedded in the model. Finally, the fused features are fed into the fully connected layer for detection. Comparison results with other network models show that the proposed model achieves an F1 score of 99.33%, which is an improvement of 0.61%~4.10%, while maintaining a lower computational cost, with FLOPs of only 1.047 M and model parametric quantities of only 69.09 M.
Han Wenxu , Zhang Xiangfeng , Jiang Hong , Jiao Wenbo , Gao Bo
2025, 48(4):44-50.
Abstract:In order to solve the situation that the gray wolf algorithm is easy to fall into the local extreme value during the path planning process, this paper proposes an improved gray wolf algorithm incorporating the artificial potential field. Firstly, the convergence factor is improved by a nonlinear strategy to ensure the diversity of populations in the search process; secondly, the gray wolf position update strategy is improved by combining with the beetle antennae search strategy update method to improve the searching ability of the algorithm; finally, the gray wolf algorithm and artificial potential field are fused to improve the searching efficiency of the algorithm as well as the security of the paths. The experimental results show that the improved gray wolf algorithm reduces; the number of robot steering while ensuring a shorter path length.
Huang He , Zou Shuai , Yang Jing , Huang Fuchuan
2025, 48(4):51-61.
Abstract:In the grinding system of heavy calcium carbonate, particle size was esteemed as a crucial metric for assessing product quality. The precise prediction of particle size was deemed instrumental in controlling product quality and informing the adjustments of vertical roller mill (VRM) parameters. Therefore, the study proposed a model for predicting the particle size of heavy calcium powder, which combined the bidirectional time convolutional network (BiTCN) based on the ivy algorithm (IVYA) with the bidirectional gated recurrent unit (BiGRU). Initially, feature and label data were subjected to preprocessing, employing time-varying filtering empirical mode decomposition in conjunction with wavelet thresholding to eliminate high-frequency noise from the primary current of the vertical mill. Then, the correlations between multidimensional features in the time series were explored from both forward and backward directions through BiTCN. At the output end of BiGRU, an attention module was incorporated to assign different weights to each position, thereby effectively focusing on the key data in the sequence. Finally, the IVYA was integrated into the overall model to ascertain the optimal solutions for critical hyperparameters within the neural network. Actual measured data from a carbonate powder mill factory were subsequently employed to simulate particle size prediction. The experimentation indicates that the optimized model using the IVYA exhibits superior predictive performance compared to other single and combined models. Specifically, it achieves the following metrics: root mean square error of 0.824 4, mean absolute error of 0.423 0, mean relative percentage error of 1.295 4%, and coefficient of determination of 98.95%.
Yang Xuhong , Yu Jiawei , Zhang Sujie , Qian Fengwei
2025, 48(4):62-70.
Abstract:Proton exchange membrane fuel cell (PEMFC) has problems such as unstable output voltage and low power generation efficiency, so Boost circuit is needed to ensure voltage quality and meet system requirements. According to the output characteristics of PEMFC, the mathematical models of PEMFC and Boost circuit are built on the Matlab/Simulink platform. Considering that linear active disturbance rejection control (LADRC) has excellent dynamic response speed to disturbance, a fuzzy neural network-linear active disturbance rejection control (FNN-LADRC) is proposed for voltage loop control of Boost circuits. The key parameters of the linear active disturbance rejection controller are tuned by FNN-LADRC to realize real-time optimization of the controller. The simulation analysis compares the performance difference of output voltage between the FNN-LADRC control strategy and the LADRC control strategy under different working conditions. The results show that the regulation time under the FNN-LADRC control strategy is 5 ms and the regulation time under the LADRC control strategy is 40 ms without disturbance. The FNN-LADRC control strategy has faster adjustment time and stronger anti-interference ability under disturbed conditions. Combined with IAE index and ITAE index, the effectiveness and superiority of the proposed control strategy are verified.
Li Junyi , Wang Xingxing , Chen Xiang , Chen Linfei , Deng Yelin
2025, 48(4):71-79.
Abstract:To provide accurate and reliable prediction of the state of health of lithium batteries, a prediction model based on the African Vulture Optimization Algorithm fused with the Aquila Optimizer to optimize the BP neural network is proposed. Through the analysis of voltage, current and temperature data during the battery charging process, the correlation between the health factors and the SOH of the battery is verified based on grey correlation analysis, and four health factors are identified as inputs to the model, which are combined with the BP neural network model based on AO-AVOA optimization to achieve a more accurate SOH prediction. The proposed model is compared with other optimization models for SOH prediction of lithium batteries, and the results show that the average absolute error of less than 0.008 9, the root mean square error below 0.011 2, and the average absolute percentage error under 1.451 2%. This model is characterized by high accuracy and robust generalization capabilities, making it highly effective for SOH prediction in lithium batteries.
2025, 48(4):80-90.
Abstract:Considering the characteristics of the interaction in the current volleyball group behavior recognition methods, such as directed, delay and subject to spatio-temporal constraints, this paper proposes a volleyball group behavior recognition method considering multi-scale spatio-temporal causal features. Firstly, the causal relationship of behavior interaction was analyzed and judged based on the causal detection model. Secondly, based on the temporal causality diagram reasoning model, the temporal causal features in crowd behavior were extracted to reduce the error caused by the delay pair feature fusion process. Then, the distance and appearance constraints are introduced into the temporal causal features to extract the multi-scale spatio-temporal causal features of crowd behavior. Finally, the effectiveness of the proposed algorithm is verified by public datasets and self-selected datasets, and the importance of the modules in the recognition framework is verified by fusion and comparison experiments. The experimental results show that the proposed model can give full play to the advantages of multi-scale spatio-temporal causal maps in describing group interaction, and the extracted multi-scale spatio-temporal causal feature maps can effectively learn the characteristics of crowd behavior. It can improve the performance of volleyball group behavior recognition.
Liu Chao , Ye Huasheng , Shen Yue , Liu Hui , Shen Yayun
2025, 48(4):91-100.
Abstract:To solve the problem of difficulty in obtaining the parameters of the dynamic model of underactuated unmanned surface vehicle, and the tracking control is susceptible to interference from water flow and natural wind, this paper proposes a trajectory tracking control method based on adaptive time delay estimation and super-twisting fast terminal sliding mode. First, the hand position point coordinate transformation method is used to reconstruct the state expansion of the underactuated unmanned surface vehicle mathematical model; then adaptive time delay estimation technology is used to estimate the unknown dynamic model part of the unmanned surface vehicle and external disturbances, and design a super-twisting fast terminal sliding mode control strategy compensates for errors caused by time delay estimation and improves the robustness of the control system; finally, the feasibility of the control method in this study is verified based on simulation analysis and navigation tracking experiments. In the trajectory tracking experiment, the average positional error was reduced by 35.1%, 24.7%, and 20.8% compared to the PID algorithm, sliding mode algorithm, and adaptive delay estimation sliding mode algorithm, respectively, demonstrating high trajectory tracking accuracy. The control method proposed in this paper does not rely on the parameters of the dynamic model and can achieve precise trajectory tracking control for underactuated unmanned surface vehicles. It features fast convergence speed and high robustness, providing a reference method for trajectory tracking control of underactuated unmanned surface vehicles.
Cao Rui , Tian Hanmin , Tian Xuemin , Ma Xulei , Liu Weilong
2025, 48(4):101-107.
Abstract:Aiming at the problem of limited measurement data and relatively low detection accuracy of planar array sensors with 16 and below electrode plates in the capacitance tomography system, as well as the complexity of three-level control of 64 electrode plates, a capacitance tomography system based on 64 electrode two-level control dense planar array sensors has been developed. Through hardware two-level line selection control and software sensitive electrode measurement method, the speed and accuracy of capacitance tomography imaging have been significantly improved. Theoretical calculations and experimental results show that the capacitance tomography imaging accuracy of the 64 electrode dense planar array sensor with secondary control reaches over 95%, and the speed reaches about 2 s, proving the feasibility and effectiveness of this design in the field of non-destructive testing of composite materials.
Chen Guo , Wang Xuemei , Ni Wenbo , Wu Senlin
2025, 48(4):108-117.
Abstract:To address the limitations of traditional ultrasonic nondestructive testing techniques, which often have detection blind spots and cannot simultaneously detect both surface and internal defects in test specimens, a novel Electromagnetic Acoustic Transducer was designed based on the principles of electromagnetic ultrasonics. This EMAT can simultaneously generate Rayleigh and shear waves, enabling concurrent detection of surface and internal defects in specimens. A rayleigh-bulk wave composite EMAT simulation model was developed in COMSOL to analyze the generation mechanisms of the composite ultrasonic waves and their interactions with defects. The composite EMAT was designed and fabricated, and an electromagnetic ultrasonic signal detection system was established. Detection and localization experiments were conducted on custom aluminum alloy specimens containing surface and internal defects. The results indicate that the designed rayleigh-bulk wave composite EMAT effectively detects both surface and internal defect echoes, achieving a localization error of 0.48% for surface defects and 0.7% for internal defects.
2025, 48(4):118-127.
Abstract:Aiming at the problem of low accuracy in object detection caused by complex background, large scale changes, and dense small targets in remote sensing images, an improved YOLOv8n detection algorithm, MGL-YOLO, is proposed. Firstly, design MSConv to reduce model parameters and reconstruct C2f module based on MSConv to improve multi-scale feature extraction capability; secondly, based on GLSA and GSConv modules, the BiFPN structure is improved to simplify the neck network and enhance its feature processing capability. Design a Light head model at the head to further reduce weight and enhance the ability to extract small target features; finally, the NWD loss function is introduced to replace the original loss function and enhance the localization accuracy for small targets. Verified on the DIORR, DOTAv1.0, and VEDAI datasets, the experimental results show that the MGL-YOLO model has high accuracy on the DIOR-R dataset mAP@0.5 Improved by 1.7% and 1.3% compared to the benchmark model, 1.0% and 1.1% on the DOTAv1.0 dataset, and 2.4% and 2.1% on the VEDAI dataset. The parameter count has been reduced by 47%, and the computational complexity has been reduced by 32%. Compared with other small object detection algorithms, it has also achieved good detection performance.
Gan Yuanping , Huang Chao , Miao Luzhen , Gao Bingpeng , Cheng Zhideng
2025, 48(4):128-138.
Abstract:As an important means to detect mine safety, ground sound monitoring technology is receiving more and more attention. In order to achieve de-noising of mine acoustic emission signals, a noise reduction method based on improved symplectic geometric mode decomposition (SGMD) combined with wavelet threshold (WT) is proposed to address the uncertainty of analysis results of SGMD. Firstly, the symplectic geometric modal components (SGCs) decomposed by SGMD are screened by setting the energy entropy increment and frequency correlation number threshold, and the active and noise components of the signal are obtained. The effective signal mode is denoised and reconstructed by wavelet threshold, and the original signal is denoised. In order to verify the effectiveness and robustness of the proposed method, the model is experimentally studied by using simulated signals and measured signals. The experimental results show that the proposed method is less timeconsuming, the highest SNR is 27.2 dB, and the smallest RMSE is 0.039. The noise reduction effect is obviously better than other noise reduction methods.
Shen Zhe , Chen Haifeng , Wang Yuanhui , Qu Zeng , Yu Hang
2025, 48(4):139-148.
Abstract:The current lithium battery state of health (SOH) estimation technology primarily focuses on non-life-supporting devices such as electric vehicle power batteries. When applied to artificial heart pump lithium batteries, significant operational differences and the inability of simple models to characterize complex electrochemical reactions limit the accuracy and reliability of SOH estimation. To address the inherent contradiction between the computational complexity of higher-order models and the accuracy of SOH assessment for artificial heart pump lithium batteries, a self-optimizing key health factor estimation algorithm is proposed to establish a lithium battery model. Firstly, to tackle the issue of inaccurate impedance measurement due to the nonlinear currentvoltage characteristics of lithium battery electrochemical systems, a quasi-steady-state electrochemical impedance spectroscopy method is designed, and a self-developed EIS testing device is used to obtain multidimensional impedance information under various temperatures, state of charge, and frequencies. Then, the linear relationship between impedance information and battery health status is analyzed, a minimized impedance objective function is established, and an improved particle swarm optimization algorithm is utilized to solve the optimization problem. Finally, hardware-in-the-loop simulation experiments simulate different conditions under the pulsating mode of artificial heart pump lithium batteries and validate the feasibility and effectiveness of the proposed method. Experimental results show that the proposed algorithm has an estimation error of less than 2% for key health factors under different SOC and temperatures; compared to the standard PSO algorithm, the estimation accuracy of the proposed algorithm is increased by 1.88%, meeting the high-precision model establishment requirements and SOH estimation for artificial heart pump lithium batteries.
Xu Jiayu , Zheng Zhanheng , Zeng Qingning , Wang Jian
2025, 48(4):149-157.
Abstract:In order to solve the problems of insufficient extraction of key speech features and single model structure, a double-stage speech enhancement method incorporating multi-scale features and improved gated Conformer was proposed to solve the problems of insufficient extraction of key features of speech and single model structure. Firstly, in order to solve the problem of insufficient extraction of key features, a two-channel convolutional fusion module was proposed, which used two-dimensional convolutional multi-scale extraction of speech key information with different receptive fields, and combined with the gating mechanism to enhance the short-term and long-term sequence correlation of the network, so as to improve the speech enhancement effect of the model in complex environments. An improved Conformer is proposed, which uses time attention and frequency attention to model in the time and frequency domains respectively, and combines the dilated convolution module to efficiently extract local and global context information, so as to enhance the performance ability of the network in speech sequence modeling. Secondly, for the problem with a single model structure, a two-stage processing structure is adopted to deal with the complex problem step by step. In the first stage, the amplitude of the noise spectrum is received, the amplitude of the clean speech is preliminarily estimated, and the noise phase is reconstructed to obtain the rough complex spectrum. In the second stage, on the basis of the rough spectrum obtained in the first stage, more refined features were further extracted to enhance the detailed expression ability of the speech signal. Finally, the experimental results are carried out on the VoiceBank+DEMAND dataset, and the experimental results show that the objective evaluation index and short-term intelligibility of this model are increased by 50.25% and 3.26%, respectively, compared with the noisy voice, indicating that the proposed algorithm can improve the intelligibility of speech more effectively, and at the same time improve the overall quality of speech signals, and has strong noise reduction ability.
Wu Ge , Zhu Yufan , Ye Tiancheng
2025, 48(4):158-168.
Abstract:Aiming at the issues of outdated existing models for steel surface defect detection, limited accuracy, and the existence of misjudgment and omission, an improved YOLOv10 method for steel surface defect detection, named FAA-YOLO, is proposed. This method introduces the lightweight network FasterNet and multi-scale attention mechanism EMA, designing the C2f_Faster_EMA module to balance the lightweight of the backbone network with enhanced feature extraction capabilities. An adaptive fine-grained channel attention mechanism AFGC is added at the end of the backbone network to enhance the preliminary feature extraction ability of the model′s backbone network, thereby improving the model′s detection accuracy. The Neck part is replaced with an attention scale sequence fusion framework ASF to enhance the model′s ability to integrate multi-scale feature information. Comparative experiments and ablation experiments on the NEU-DET steel surface defect dataset show that the proposed FAA-YOLO model reduces the number of parameters by 11.01%, the computational load by 7.69%, and increases the detection accuracy by 2.9 percentage points, achieving a detection accuracy of 83.6%. This method reduces the complexity of the model while achieving high detection accuracy, demonstrating high usability and real-time performance in industrial systems.
Yang Luxia , Xue Yingzhao , Zhang Hongrui , Ma Yongjie
2025, 48(4):169-180.
Abstract:Addressing the issue of low vehicle recognition accuracy stemming from dense vehicle targets and occlusions in complex traffic scenarios, a vehicle recognition algorithm utilizing highorder spatial feature aggregation is proposed. Initially, during the downsampling phase of feature extraction, the HSIDM module is devised to facilitate deeper feature aggregation and minimize the loss of fine details. Subsequently, within the feature fusion component, the DMFAM module is introduced to dynamically adjust the weights of features across various scales, thereby acquiring multi-scale contextual information and bolstering the model′s adaptability to diverse features. Following this, a decoupled REL-Head detector is formulated to disentangle classification and regression tasks, preventing task mixing and enhancing the learning capability and interference resistance of local features. Ultimately, the model presented in this paper is deployed on edge devices for testing. Experimental outcomes reveal that on the complex traffic scene datasets BIT-Vehicle and UA-DETRAC, the mean average precision (mAP) of our algorithm has improved by 0.7% and 3.9% respectively compared to the original model. Additionally, it operates seamlessly on edge devices, demonstrating effective recognition capabilities. This indicates that the proposed approach can effectively enhance the precision of vehicle identification and is suitable for use on constrained devices.
2025, 48(4):181-190.
Abstract:In end-side production line industrial quality inspection applications for large-scale manufacturing, tailoring and deploying deep learning models to edge devices with small arithmetic power becomes particularly important due to the limitations of arithmetic power, cost and power consumption. Based on the application scenario of complex defect detection of aluminum profile, the defect detection model is designed based on YOLOv8. First of all, through the lightweight structure design, combined with the partial self-attention mechanism to improve the ability of subtle defect extraction; the use of spatial channel downsample instead of the traditional downsampling convolution; and proposed a combination of mixed local channel attention mechanism of the C2f-M module. Then, SC-BiFPN neck network is designed based on bidirectional feature pyramid network, which enhances the multi-scale feature fusion capability. Then, the task dynamic align detection head is designed to make full use of multilevel features for more accurate target localisation and classification; the MPDIoU loss function is used to enhance the bounding box regression capability. Finally, YOLOv8 is trimmed by Taylor's method to significantly reduce the number of model parameters and computational cost. The experimental results show that the lightweight YOLOv8 model reduces the number of parameters to 36.7% of the original model on the aluminium surface defects dataset, reduces the computational effort by 40%, and reduces the model volume by 62%; at the same time, the detection accuracy, the recall rate and the mAP@50.95 are improved by 0.3%, 1.1%, and 4.8%, respectively. The method effectively solves the problem of balancing computational complexity and detection performance in end-side deployment, and provides a feasible solution for efficient defect detection on small arithmetic hardware.
2025, 48(4):191-198.
Abstract:3D object detection is a critical technology for automatic driving perception systems, which accurately detects the state of the driving environment and ensures the safety of driving. Aiming at the problem of low 3D detection accuracy of small objects such as pedestrians and cyclists, a 3D object detection algorithm based on multi-scale features and adaptive non-maximum suppression (ANMS) is proposed. Firstly, a multi-scale feature extractor is designed to obtain large, medium, and small-sized features. Secondly, a multi-scale detection head is constructed to generate the candidate boxes of objects of different sizes, thereby supplementing the candidate boxes of small objects. To balance the number of multi-scale candidate boxes, a candidate box screening algorithm on ANMS is designed, enhancing the detection accuracy of objects of different sizes. The results on the KITTI dataset indicate that the improved algorithm achieves 62.57% and 73.30% detection accuracy for pedestrians and cyclists, which is 2.04% and 1.33% higher than the baseline while ensuring the detection accuracy of car-type objects, which verifies that the improved algorithm has preferable 3D detection performance in small object detection.
Editor in chief:Prof. Sun Shenghe
Inauguration:1980
ISSN:1002-7300
CN:11-2175/TN
Domestic postal code:2-369